Multi-task sparse Bayesian extreme learning machine regression method
An extreme learning machine and sparse Bayesian technology, applied in the field of multi-task sparse Bayesian extreme learning machine regression, can solve the problem of overfitting, extreme learning machine performance depends on the number of hidden layer neurons, theoretical research Issues such as less development
- Summary
- Abstract
- Description
- Claims
- Application Information
AI Technical Summary
Problems solved by technology
Method used
Image
Examples
Embodiment
[0177] This implementation mode is to apply the present invention to the regression problem of the parameter relationship for cohesive soil in geotechnical engineering. figure 1 A flow chart of the method of the present invention is given. figure 2 The neural network architecture and solution mode of the sparse Bayesian extreme learning machine in the present invention are given. image 3 , Figure 4 The application advantages and application effects of the present invention in the simulated data set are given.
[0178] Aiming at the CLAY data set in the ISSMGE-TC304 database, the five physical quantities of cohesive soil, namely liquidity index, vertical effective stress, pre-consolidation stress, undrained shear strength and drained shear strength, are used as objects to study the regression among parameters The relationship is as follows:
[0179] The first step is as follows: for task 1, the liquidity index and the vertical effective stress are used as input, and the l...
PUM
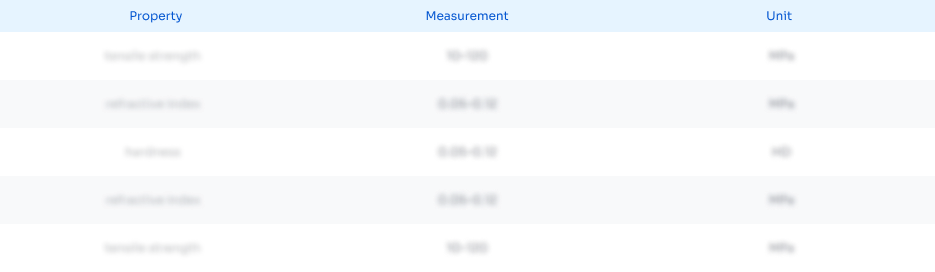
Abstract
Description
Claims
Application Information
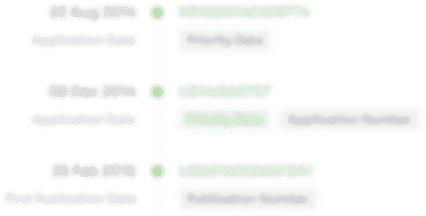
- R&D Engineer
- R&D Manager
- IP Professional
- Industry Leading Data Capabilities
- Powerful AI technology
- Patent DNA Extraction
Browse by: Latest US Patents, China's latest patents, Technical Efficacy Thesaurus, Application Domain, Technology Topic, Popular Technical Reports.
© 2024 PatSnap. All rights reserved.Legal|Privacy policy|Modern Slavery Act Transparency Statement|Sitemap|About US| Contact US: help@patsnap.com