Improved YOLO-V3 metal processing surface defect detection method
A metal processing and defect detection technology, applied in image analysis, image enhancement, instruments, etc., can solve the problems of poor detection effect, inability to accurately identify small objects, low recall rate, etc., and achieve accurate detection and reduce the downsampling factor Effect
- Summary
- Abstract
- Description
- Claims
- Application Information
AI Technical Summary
Problems solved by technology
Method used
Image
Examples
Embodiment Construction
[0045] The present invention will be further described in detail below in conjunction with the accompanying drawings.
[0046] Such as figure 1 As shown, it is a flow chart of a specific implementation of an improved YOLO-V3 metal processing surface defect detection method disclosed in the present invention. In this embodiment, the improved YOLO-V3 includes a feature extraction backbone network . A multi-scale fusion module and a multi-classifier module, wherein the metal processing surface defect detection method includes:
[0047] S1. Acquiring metal surface processing images;
[0048]S2. Input the metal surface processing image into the feature extraction backbone network to extract feature maps of different scales. The feature extraction backbone network has no pooling layer and the downsampling factor is smaller than that of Darknet-53, and the number of convolutional layers is less than that of Darknet. -53 convolutional layers;
[0049] Since the pixel area of the...
PUM
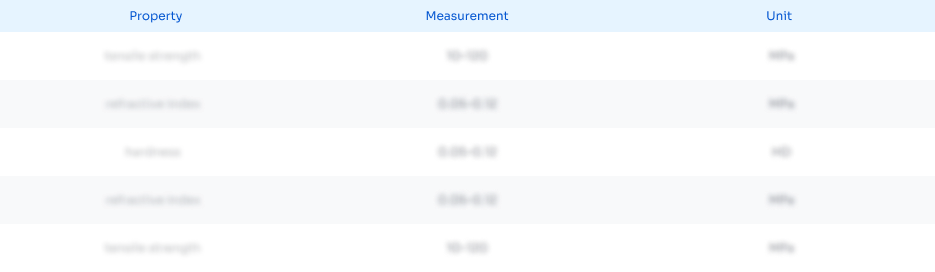
Abstract
Description
Claims
Application Information
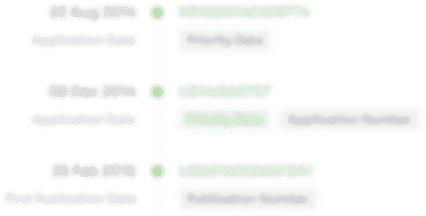
- R&D Engineer
- R&D Manager
- IP Professional
- Industry Leading Data Capabilities
- Powerful AI technology
- Patent DNA Extraction
Browse by: Latest US Patents, China's latest patents, Technical Efficacy Thesaurus, Application Domain, Technology Topic, Popular Technical Reports.
© 2024 PatSnap. All rights reserved.Legal|Privacy policy|Modern Slavery Act Transparency Statement|Sitemap|About US| Contact US: help@patsnap.com