Spectral baseline correction method, system and detection method in tea near-infrared spectral analysis
A near-infrared spectroscopy and baseline correction technology, which is applied in the field of spectral baseline correction in the near-infrared spectroscopy analysis of tea, can solve the problems of under-fitting in weight update, inaccurate correction results, and failure to consider error trends, and achieve a wide range of parameter selection. , reduce the optimization process, and avoid the effect of untimely weight update
- Summary
- Abstract
- Description
- Claims
- Application Information
AI Technical Summary
Problems solved by technology
Method used
Image
Examples
Embodiment 1
[0070] like figure 1 As shown, the present embodiment provides a method of calculating the spectrum of tea near infrared spectroscopy, in particular:
[0071] Step 1: Data acquisition and parameter initialization; collecting green tea spectrum data is ( figure 2 ), The sugar content data is The initialization parameter is set to: smooth coefficient λ = 10 4 , Number of iterations T = 30, balance coefficient α = 60, weight coefficient W 0 = [1, 1, ..., 1] and relative fitting error coefficient δ = 10 -3 , Fit baseline differential matrix:
[0072]
[0073] Step 2: Calculate the fitting baseline; establish a penalty least squares optimization function based on the analysis data and initialization parameters:
[0074] J = (Y-Z) T W (y-z) + λz T Di T DZ (12)
[0075] Further, the equation (12) is further deflected, and the calculation formula based on the weight coefficient calculation of the calculation of the proposed baseline:
[0076] z = (w + λd T D) -1 WY (13)
[0077] Step ...
Embodiment 2
[0097] Compared with Embodiment 1, the present embodiment provides a spectral baseline correction system during a tea near infrared spectroscopy, including:
[0098] Tea sample collection module: collecting tea samples, gets tea near infrared spectroscopy, forming raw data; data acquisition and parameter initialization module: collecting green tea spectral data ( figure 2 ), The sugar content data is The initialization parameter is set to: smooth coefficient λ = 10 4 , Number of iterations T = 30, balance coefficient α = 60, weight coefficient W 0 = [1, 1, ..., 1], and relative fitting error coefficient Δ = 10 -3 , Fit baseline differential matrix
[0099]
[0100] Calculate the fitting baseline module: according to the penalty minimum multiplier, based on the equipped baseline calculated by the weight coefficient;
[0101] Correction error module: calculate the difference between the original data and the fitting baseline;
[0102] Interval Confirmation Module: Determine the ...
PUM
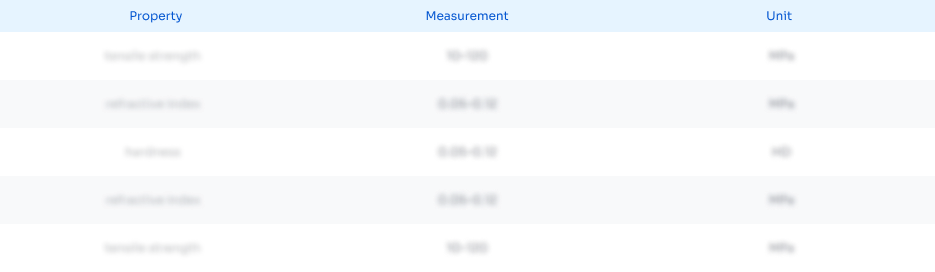
Abstract
Description
Claims
Application Information
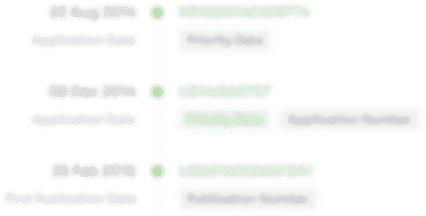
- R&D Engineer
- R&D Manager
- IP Professional
- Industry Leading Data Capabilities
- Powerful AI technology
- Patent DNA Extraction
Browse by: Latest US Patents, China's latest patents, Technical Efficacy Thesaurus, Application Domain, Technology Topic, Popular Technical Reports.
© 2024 PatSnap. All rights reserved.Legal|Privacy policy|Modern Slavery Act Transparency Statement|Sitemap|About US| Contact US: help@patsnap.com