Single motor imagery electroencephalogram signal recognition method based on multi-linear principal component analysis
A principal component analysis, single-motion technology, applied in the direction of biometric recognition mode, biometric recognition, character and pattern recognition based on physiological signals, etc., can solve the problem of ignoring the frequency domain information of EEG signals, relying on wavelet transform effect, and insufficient effect. Stability and other issues, to achieve the effect of high EEG recognition effect
- Summary
- Abstract
- Description
- Claims
- Application Information
AI Technical Summary
Problems solved by technology
Method used
Image
Examples
Embodiment Construction
[0038]Hereinafter, embodiments of the present invention will be described with reference to the drawings.
[0039] The overall flow chart of a single motor imagery EEG signal recognition method based on multi-linear principal component analysis proposed by the embodiment of the present invention is as follows figure 1 As shown, the method includes the following steps:
[0040] Step 1, use the wavelet analysis method to establish the third-order EEG tensor data of multiple experiments, and randomly divide it into a training set and a test set, including the following specific steps:
[0041] Step 11. When the subject imagines the movement of the left and right hands according to the prompt, the high-precision mobile brain wave testing instrument EMOTIV EPOC+14 is used to collect the subject's EEG data, and the collected continuous EEG signal is intercepted according to the time point of the prompt The EEG data of each channel of each subject's motor imagery is formed, and fina...
PUM
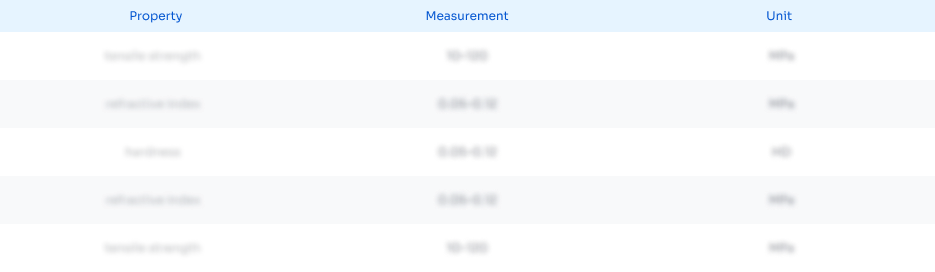
Abstract
Description
Claims
Application Information
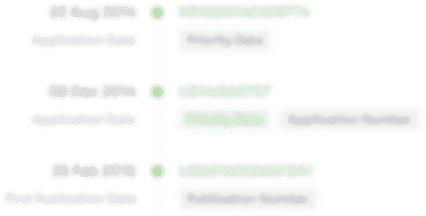
- R&D Engineer
- R&D Manager
- IP Professional
- Industry Leading Data Capabilities
- Powerful AI technology
- Patent DNA Extraction
Browse by: Latest US Patents, China's latest patents, Technical Efficacy Thesaurus, Application Domain, Technology Topic, Popular Technical Reports.
© 2024 PatSnap. All rights reserved.Legal|Privacy policy|Modern Slavery Act Transparency Statement|Sitemap|About US| Contact US: help@patsnap.com