Decomposition-based high-dimensional multi-objective evolution method
A multi-objective evolutionary, high-dimensional technology, applied in the field of intelligent optimization algorithms, can solve problems such as difficult convergence and diversity
- Summary
- Abstract
- Description
- Claims
- Application Information
AI Technical Summary
Problems solved by technology
Method used
Image
Examples
Embodiment 1
[0093] Embodiment 1: as Figure 1-10 As shown, a decomposition-based high-dimensional multi-objective evolutionary algorithm includes steps 1-8.
[0094] Step 1, parameter setting: algebra g=0, maximum number of iterations G max , the target number M≥4, and the population size N.
[0095] Step 2, generate N reference vectors W={W 1 ,W 2 ,...,W N}:
[0096] Step 2.1, using the Normal Boundary Intersection (PBI) method to generate uniformly distributed reference points RP on a unit hyperplane L = {RP 1 ,RP 2 ,...,RP N};
[0097] Step 2.2, based on the reference point RP 1 ,RP 2 ,...,RP N Construct a uniformly distributed reference vector W in the target space 1 ,W 2 ,...,W N . A reference vector satisfies the following conditions:
[0098]
[0099] in Indicates the reference vector W i The component values of the j-th dimension of and H means that each target is divided into H equal parts, and the number of generated reference vectors is N, where Such...
PUM
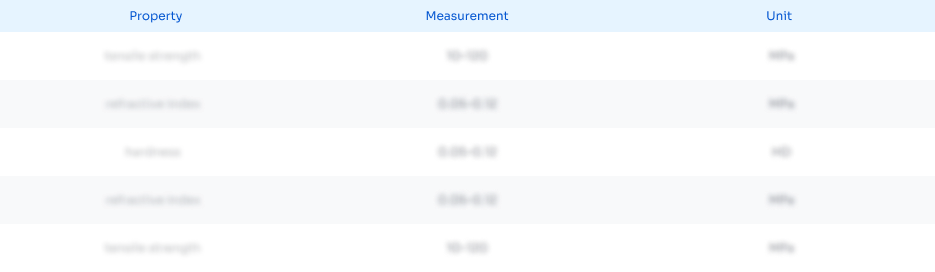
Abstract
Description
Claims
Application Information
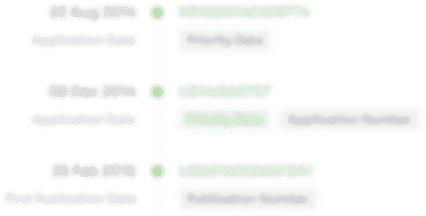
- R&D Engineer
- R&D Manager
- IP Professional
- Industry Leading Data Capabilities
- Powerful AI technology
- Patent DNA Extraction
Browse by: Latest US Patents, China's latest patents, Technical Efficacy Thesaurus, Application Domain, Technology Topic.
© 2024 PatSnap. All rights reserved.Legal|Privacy policy|Modern Slavery Act Transparency Statement|Sitemap