Adversarial sample generation method based on depth product quantization
A technique of adversarial samples and products, applied in the field of artificial intelligence, can solve the problems of discrete quantization process of deep product quantification retrieval system
- Summary
- Abstract
- Description
- Claims
- Application Information
AI Technical Summary
Problems solved by technology
Method used
Image
Examples
Embodiment Construction
[0033] In order to make the object, technical solution and advantages of the present invention more clear and definite, the present invention will be further described in detail below with reference to the accompanying drawings and examples. It should be understood that the specific embodiments described here are only used to explain the present invention, not to limit the present invention.
[0034] Various non-limiting embodiments of the present invention will be described in detail below in conjunction with the accompanying drawings.
[0035] This embodiment provides a method for generating adversarial samples based on depth product quantization, such as figure 1 As shown, the adversarial sample generation method based on depth product quantization includes:
[0036] S10. Input the original image into a preset network model, so as to output a quantization distribution center vector corresponding to the original image through the preset network model.
[0037] Specifically...
PUM
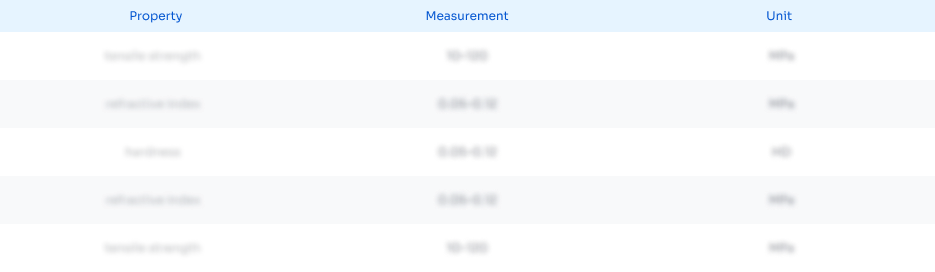
Abstract
Description
Claims
Application Information
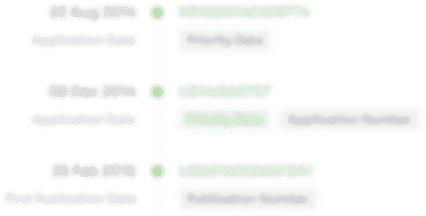
- R&D Engineer
- R&D Manager
- IP Professional
- Industry Leading Data Capabilities
- Powerful AI technology
- Patent DNA Extraction
Browse by: Latest US Patents, China's latest patents, Technical Efficacy Thesaurus, Application Domain, Technology Topic, Popular Technical Reports.
© 2024 PatSnap. All rights reserved.Legal|Privacy policy|Modern Slavery Act Transparency Statement|Sitemap|About US| Contact US: help@patsnap.com