Alzheimer's disease classification method and system based on anatomical landmark and residual network
An Alzheimer's disease and classification method technology, applied in the field of Alzheimer's disease classification methods and systems, can solve the problems of not taking into account subtle changes, high time and cost, low classification accuracy, etc., to achieve auxiliary The effect of clinical diagnosis, reducing time cost, avoiding the risk of overfitting
- Summary
- Abstract
- Description
- Claims
- Application Information
AI Technical Summary
Problems solved by technology
Method used
Image
Examples
Embodiment 1
[0035] Such as figure 1 As shown, this embodiment provides a classification method for Alzheimer's disease based on anatomical landmarks and residual networks, including:
[0036] Step 1. After tissue segmentation and modulation of the training image set, the gray matter image is obtained;
[0037] Step 2. Perform a two-sample t-test on the voxels of the Alzheimer's disease image and the normal subject image in the training image set, and identify anatomical landmarks with a preset significance level threshold;
[0038] Step 3, extracting the gray matter feature block of the gray matter image centered on the obtained anatomical landmark;
[0039] Step 4. Connect the gray matter feature blocks, input the obtained synthetic blocks into the residual network model for feature extraction, use the features extracted by the residual network model as the input of the classifier, and test the Alzheimer's disease patients in the test image set Classified with normal subjects.
[0040...
Embodiment 2
[0065] This embodiment provides an Alzheimer's disease classification system based on anatomical landmarks and residual networks, including:
[0066] The preprocessing module is configured to obtain gray matter images after tissue segmentation modulation is performed on the training image set;
[0067] The anatomical landmark acquisition module is configured to compare the voxels of the Alzheimer's disease images in the training image set and the images of normal subjects to identify anatomical landmarks;
[0068] The gray matter feature extraction module is configured to extract the gray matter feature block of the gray matter image centered on the obtained anatomical landmark;
[0069] The training and classification module is configured to connect the gray matter feature blocks, input the obtained synthetic blocks to the residual network model for feature extraction, use the features extracted by the residual network model as the input of the classifier, and use the Al of t...
PUM
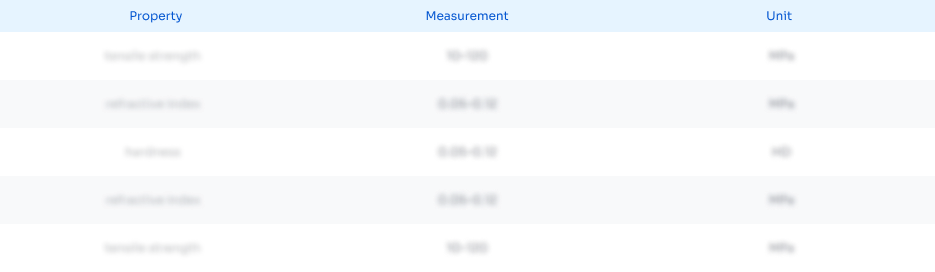
Abstract
Description
Claims
Application Information
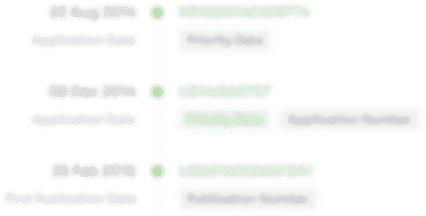
- R&D Engineer
- R&D Manager
- IP Professional
- Industry Leading Data Capabilities
- Powerful AI technology
- Patent DNA Extraction
Browse by: Latest US Patents, China's latest patents, Technical Efficacy Thesaurus, Application Domain, Technology Topic, Popular Technical Reports.
© 2024 PatSnap. All rights reserved.Legal|Privacy policy|Modern Slavery Act Transparency Statement|Sitemap|About US| Contact US: help@patsnap.com