Mammary gland DCE-MRI image lesion segmentation model establishment based on hybrid convolution and segmentation method
A DCE-MRI and segmentation model technology, applied in the field of medical image analysis, can solve the problem of not being able to take into account the segmentation tasks of large and small lesions at the same time, and achieve the effect of improving the segmentation effect and improving the accuracy
- Summary
- Abstract
- Description
- Claims
- Application Information
AI Technical Summary
Problems solved by technology
Method used
Image
Examples
Embodiment Construction
[0052] A breast DCE-MRI sequence image contains n-phase images, including a phase-1 image taken before contrast agent injection and n-1-phase images taken after contrast agent injection, n is generally 7 to 9, and in the present invention, contrast injection The first-phase image taken after contrast agent injection was used as the enhanced initial image, and the last-phase image taken after contrast agent injection was used as the enhanced late image.
[0053] The enhanced peak image in the present invention refers to the first-stage image with the highest pixel average among the n-1-stage images taken after contrast agent injection.
[0054] The "three-channel image" in the present invention refers to using three single-channel images (ie silhouette image, enhanced early image and enhanced late image) as one channel of the image respectively to form an image with three channels.
[0055] The mammary gland DCE-MRI image set used in the specific embodiment of the present inven...
PUM
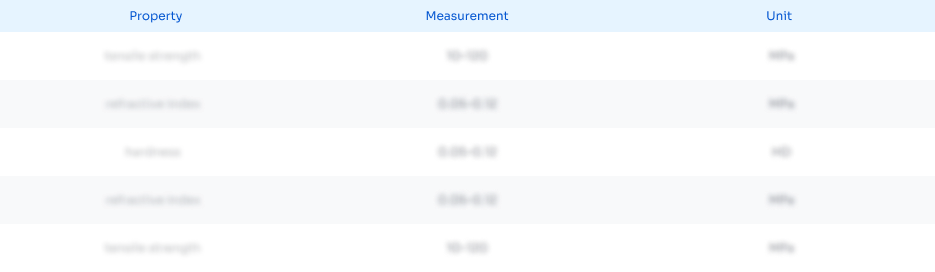
Abstract
Description
Claims
Application Information
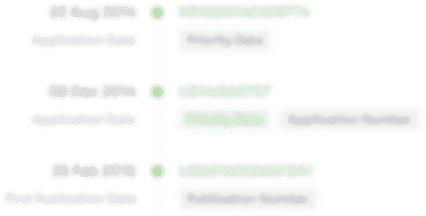
- R&D Engineer
- R&D Manager
- IP Professional
- Industry Leading Data Capabilities
- Powerful AI technology
- Patent DNA Extraction
Browse by: Latest US Patents, China's latest patents, Technical Efficacy Thesaurus, Application Domain, Technology Topic, Popular Technical Reports.
© 2024 PatSnap. All rights reserved.Legal|Privacy policy|Modern Slavery Act Transparency Statement|Sitemap|About US| Contact US: help@patsnap.com