Full-coverage task allocation method for dynamic noise environment
A task allocation and dynamic noise technology, applied in neural learning methods, machine learning, instruments, etc., can solve problems such as difficult to balance message weights, incorrect historical track record information, and incorrect environmental perception information of a single robot.
- Summary
- Abstract
- Description
- Claims
- Application Information
AI Technical Summary
Problems solved by technology
Method used
Image
Examples
Embodiment Construction
[0116] The specific implementation of the present invention will be described below by taking multi-robot dynamic noise environment rescue exploration as an example. In the present invention, the refitted wheeled mobile robot Turtlebot is selected as the executor, and a Kinect sensor, a laser ranging sensor, and a positioning device are loaded in the robot, and three collision sensors are installed on the chassis at the same time. All parts of the whole system realize data communication through LAN.
[0117] image 3 It is the overall flowchart of the present invention. Such as image 3 Shown, the present invention comprises the following steps:
[0118] In the first step, build as figure 1 The multi-robot environment shown, which consists of N (for example when experimenting with figure 1 In the shown multi-robot environment, N=4) robot nodes (wheeled mobile robot Turtlebot) and a central control node (server). The N robot nodes and the central control node are intercon...
PUM
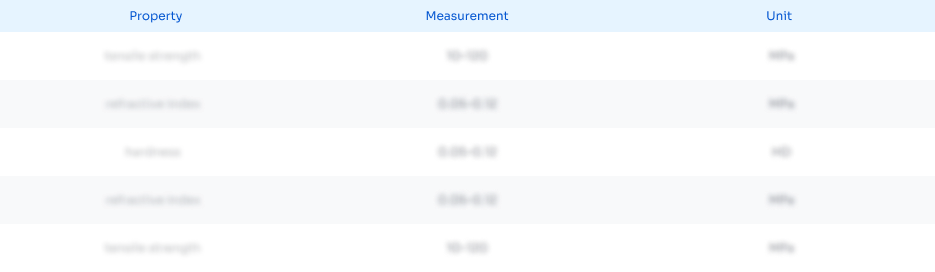
Abstract
Description
Claims
Application Information
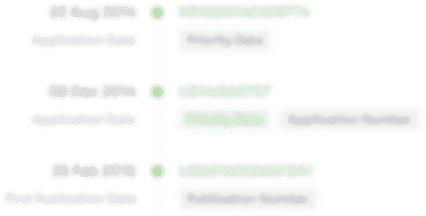
- R&D Engineer
- R&D Manager
- IP Professional
- Industry Leading Data Capabilities
- Powerful AI technology
- Patent DNA Extraction
Browse by: Latest US Patents, China's latest patents, Technical Efficacy Thesaurus, Application Domain, Technology Topic, Popular Technical Reports.
© 2024 PatSnap. All rights reserved.Legal|Privacy policy|Modern Slavery Act Transparency Statement|Sitemap|About US| Contact US: help@patsnap.com