T cell receptor sequence classification method based on semi-supervised learning framework
A semi-supervised learning, cell receptor technology, applied in the field of T cell receptor sequence classification based on semi-supervised learning framework, can solve complex experiments and other problems, and achieve the effect of helping feature extraction
- Summary
- Abstract
- Description
- Claims
- Application Information
AI Technical Summary
Problems solved by technology
Method used
Image
Examples
Embodiment Construction
[0046] The present invention provides a T cell receptor sequence classification method based on a semi-supervised learning framework, which uses a support vector machine, a random forest and a decision tree supervised learning algorithm to construct a triple learning framework to solve the aforementioned problem of less data. Support vector machines, random forests, and decision trees have their own advantages and disadvantages. First, the support vector machine has good performance on small sample sets (the amount of data will be reduced when the epitope data set is divided in different proportions), so the method can improve the accuracy of the initial classifier prediction, which helps the model The iteration improves the prediction accuracy of the final model. Random forest is not easy to overfit, and has a high tolerance for outliers and noise, and has strong robustness to unbalanced data. Decision trees are suitable for high-dimensional data and for processing samples wi...
PUM
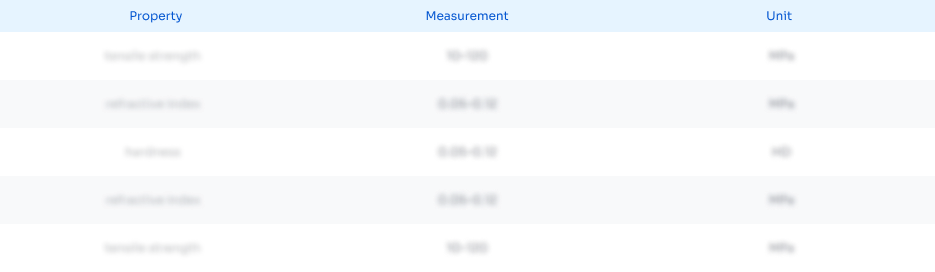
Abstract
Description
Claims
Application Information
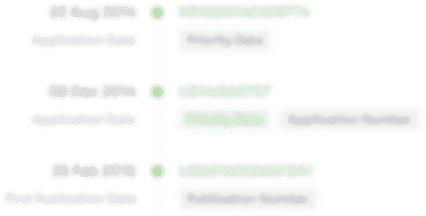
- R&D Engineer
- R&D Manager
- IP Professional
- Industry Leading Data Capabilities
- Powerful AI technology
- Patent DNA Extraction
Browse by: Latest US Patents, China's latest patents, Technical Efficacy Thesaurus, Application Domain, Technology Topic, Popular Technical Reports.
© 2024 PatSnap. All rights reserved.Legal|Privacy policy|Modern Slavery Act Transparency Statement|Sitemap|About US| Contact US: help@patsnap.com