Internet-of-Things application flow detection method based on deep learning
A technology that uses traffic and detection methods, applied in the computer field, can solve problems such as difficulties, inaccurate traffic classification, and unsatisfactory recognition accuracy, and achieve the effects of meeting security requirements, accurate identification, and ensuring credibility and security
- Summary
- Abstract
- Description
- Claims
- Application Information
AI Technical Summary
Problems solved by technology
Method used
Image
Examples
Embodiment
[0036] The invention is designed as three modules of traffic information acquisition, information structuring and cloud neural network identification, the specific process is as follows figure 1As shown, the traffic information acquisition module is the realization of step 1, including traffic acquisition and judging traffic attributes, the information structure is the realization of step 2, including traffic segmentation and structure storage, and the convolutional neural network module is the realization of step 3. Including cloud training and model checking.
[0037] The traffic information acquisition module is to set up a packet capture program on the IoT device side. By capturing the traffic packets passing through the gateway, the current traffic packet type passing through the gateway is obtained. Through the pre-set analysis program, the traffic packets are divided into application-level traffic and System-level traffic, and then through domain name resolution, applic...
PUM
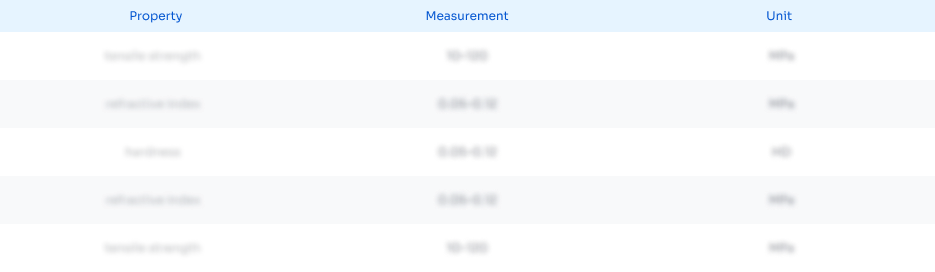
Abstract
Description
Claims
Application Information
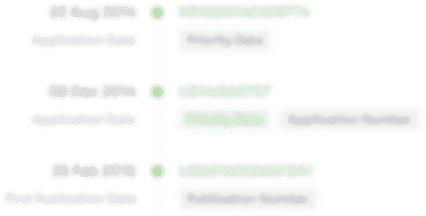
- R&D Engineer
- R&D Manager
- IP Professional
- Industry Leading Data Capabilities
- Powerful AI technology
- Patent DNA Extraction
Browse by: Latest US Patents, China's latest patents, Technical Efficacy Thesaurus, Application Domain, Technology Topic, Popular Technical Reports.
© 2024 PatSnap. All rights reserved.Legal|Privacy policy|Modern Slavery Act Transparency Statement|Sitemap|About US| Contact US: help@patsnap.com