Knowledge representation learning method fusing multi-source information
A technology of multi-source information and knowledge representation, applied in the field of knowledge representation learning that integrates multi-source information, it can solve the problems of low computational efficiency, insufficient scalability, large time and space complexity of graph theory algorithms, and achieve faster convergence. , Improve data sparseness and improve training efficiency
- Summary
- Abstract
- Description
- Claims
- Application Information
AI Technical Summary
Problems solved by technology
Method used
Image
Examples
Embodiment
[0130] parameter settings
[0131]In the encoder model, the convolutional neural network is used to obtain the features of words in the text description information, and the input dimension of words and triples is selected to be 50. The first convolutional layer window is set to 2, and the second convolutional layer window is set to 1. The nonlinear functions after the two convolutional layers all choose the tanh function. The first pooling layer uses maximum pooling with a window size of 4, and the second pooling layer uses average pooling with a window size of 1. In the hierarchical type information, each relation type and domain matrix dimension are randomly initialized to 50×50, the type matrix weight is set to 0.9, and the domain matrix weight is set to 0.1. In the first layer of GAT, two attention mechanisms are used separately, and the output dimension of each attention mechanism is 100, so the dimension of the triplet vector output after combination becomes 200, and ...
PUM
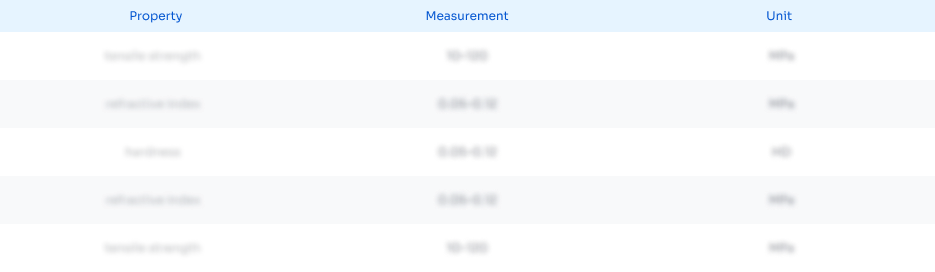
Abstract
Description
Claims
Application Information
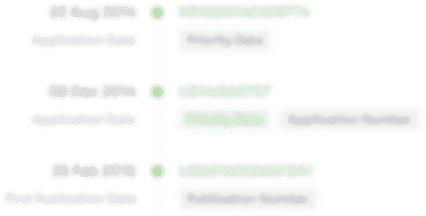
- R&D Engineer
- R&D Manager
- IP Professional
- Industry Leading Data Capabilities
- Powerful AI technology
- Patent DNA Extraction
Browse by: Latest US Patents, China's latest patents, Technical Efficacy Thesaurus, Application Domain, Technology Topic, Popular Technical Reports.
© 2024 PatSnap. All rights reserved.Legal|Privacy policy|Modern Slavery Act Transparency Statement|Sitemap|About US| Contact US: help@patsnap.com