Large-scale multi-antenna channel estimation method based on deep convolutional neural network
A deep convolution and neural network technology, applied in channel estimation, baseband system, baseband system components, etc., can solve problems such as limited accuracy and difficulty in meeting low-latency scenarios
- Summary
- Abstract
- Description
- Claims
- Application Information
AI Technical Summary
Problems solved by technology
Method used
Image
Examples
Embodiment Construction
[0026] In order to understand the technical content of the present invention more clearly, the following embodiments will further illustrate the present invention in conjunction with the accompanying drawings.
[0027] The present invention comprises the following steps:
[0028] Step 1: After having N t transmitting antennas, N r In a large-scale multi-antenna system with multiple receiving antennas, the OFDM data block of length N sent from the t-th transmitting antenna to the r-th receiving antenna is x (t) , the channel impulse response of the corresponding subchannel is h (t) , the maximum delay spread length of the channel is L. After the OFDM data block is propagated through the wireless multipath channel, the N received on the r receiving antenna corresponding to the t transmitting antenna p A normalized pilot signal is u (t) . The pilot position of the t-th transmit antenna is set by the pilot position Given, any one of the pilot subscripts is Randomly distrib...
PUM
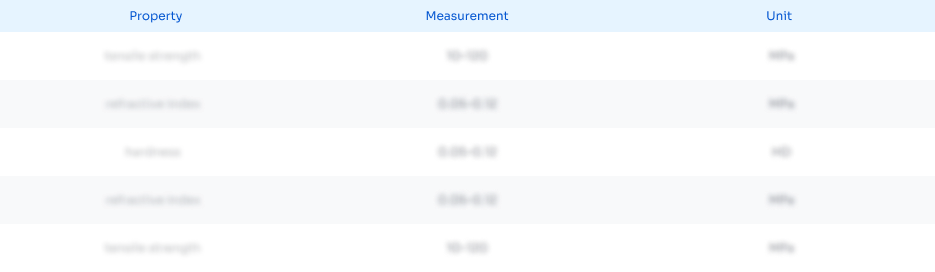
Abstract
Description
Claims
Application Information
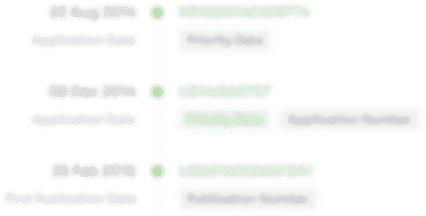
- R&D Engineer
- R&D Manager
- IP Professional
- Industry Leading Data Capabilities
- Powerful AI technology
- Patent DNA Extraction
Browse by: Latest US Patents, China's latest patents, Technical Efficacy Thesaurus, Application Domain, Technology Topic, Popular Technical Reports.
© 2024 PatSnap. All rights reserved.Legal|Privacy policy|Modern Slavery Act Transparency Statement|Sitemap|About US| Contact US: help@patsnap.com