Time series anomaly detection method and system for key performance index data
A technology for key performance indicators and anomaly detection. It is used in electrical digital data processing, error detection/correction, and response error generation. It can solve problems such as low generalization performance, unlearned training, and low detection efficiency.
- Summary
- Abstract
- Description
- Claims
- Application Information
AI Technical Summary
Problems solved by technology
Method used
Image
Examples
no. 1 example
[0068] This embodiment provides a time series anomaly detection method for KPI data. figure 1 Shown is the flow chart of the timing anomaly detection method for the KPI data. Such as figure 1 As shown, the timing anomaly detection method of the KPI data includes the following steps:
[0069] Step S1, collecting time series data of key performance indicators.
[0070] In this step, the minute-grained data of the measured object within a period of time is collected, and the data includes the following attribute fields: the sequence ID of the key performance indicator, the time stamp, and the value of the key performance indicator. Manually mark the abnormalities in the data, and the attribute field of the data will add a "label" field, with 0 indicating normal points and 1 indicating abnormal points, which are used as the basis for evaluating the model during testing. The process of manually labeling abnormalities is generally carried out by experts. This part of the work is ...
no. 2 example
[0116] This embodiment provides a timing anomaly detection system for key performance indicator (KPI) data, figure 2 A schematic diagram of the structure of the system is shown. Such as figure 2 As shown, the timing anomaly detection system for KPI data includes: a data collection module 10 , a data preprocessing module 20 , a feature splicing module 30 , a model training and testing module 40 , a model evaluation module 50 , and a final detection module 60 .
[0117] Wherein, the data collection module 10 is connected with the data preprocessing module 20 for collecting time series data of key performance indicators.
[0118] The data preprocessing module 20 is connected to the feature splicing module 30, and is used for judging missing values and abnormal values in the time series data, correcting the missing values and abnormal values, and obtaining preprocessed data.
[0119] The feature splicing module 30 is connected with the model training and testing module 4...
PUM
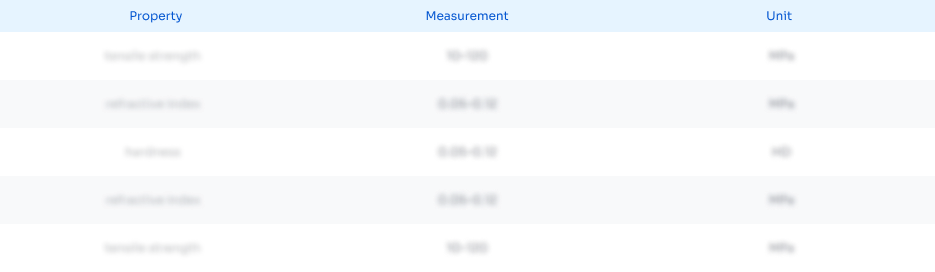
Abstract
Description
Claims
Application Information
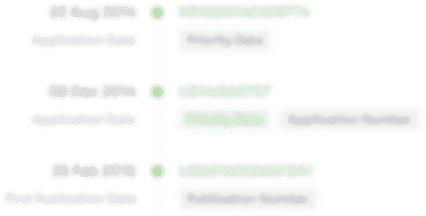
- R&D Engineer
- R&D Manager
- IP Professional
- Industry Leading Data Capabilities
- Powerful AI technology
- Patent DNA Extraction
Browse by: Latest US Patents, China's latest patents, Technical Efficacy Thesaurus, Application Domain, Technology Topic, Popular Technical Reports.
© 2024 PatSnap. All rights reserved.Legal|Privacy policy|Modern Slavery Act Transparency Statement|Sitemap|About US| Contact US: help@patsnap.com