RGB-D saliency target detection method
A RGB-D and target detection technology, applied in the field of image processing and stereo vision, can solve the problems of not being able to effectively highlight salient targets, suppress background areas, and not make full use of them, so as to achieve good salient target detection performance and improve accuracy Effect
- Summary
- Abstract
- Description
- Claims
- Application Information
AI Technical Summary
Problems solved by technology
Method used
Image
Examples
Embodiment 1
[0035] Embodiments of the present invention propose a RGB-D salient object detection method based on cross-modal joint feature extraction and low value fusion loss. By designing the cross-modal joint feature extraction part, the complementarity between RGB features and depth features is effectively captured; by designing the salient object detection part, the single-modal multi-scale features and cross-modal joint are effectively integrated feature, which improves the accuracy of saliency detection per stream; by designing a low-value fusion loss, the lower bound of the saliency value is effectively improved, and the fusion between different detection results is promoted.
[0036] The whole process is divided into six parts: 1) single-modal feature extraction; 2) cross-modal joint feature extraction; 3) salient target detection; 4) low-value fusion loss design; 5) overall network loss design; 6) network training Strategy design, the specific steps are as follows:
[0037] 1. ...
Embodiment 2
[0081] figure 1 The technical flow chart of the present invention is given, mainly including six parts: single-modal feature extraction, cross-modal joint feature extraction, salient target detection, low-value fusion loss design, overall network loss design and network training strategy design.
[0082] figure 2 A specific implementation block diagram of the present invention is given.
[0083] image 3 The structural diagrams of the cross-modal feature extraction module (CFM) and the RGB saliency detection part of the fusion block (FB) are given.
[0084] Figure 4 An example of RGB-D salient object detection is given. Among them, the first column is the RGB image, the second example is the depth map, the third column is the truth map of the salient object detection, and the fourth column is the result obtained by the method of the present invention.
[0085] It can be seen from the results that the method of the present invention effectively fuses the information of t...
PUM
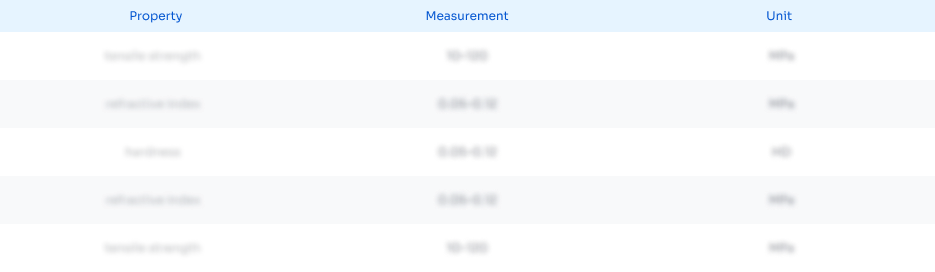
Abstract
Description
Claims
Application Information
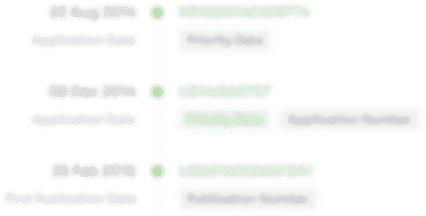
- R&D Engineer
- R&D Manager
- IP Professional
- Industry Leading Data Capabilities
- Powerful AI technology
- Patent DNA Extraction
Browse by: Latest US Patents, China's latest patents, Technical Efficacy Thesaurus, Application Domain, Technology Topic.
© 2024 PatSnap. All rights reserved.Legal|Privacy policy|Modern Slavery Act Transparency Statement|Sitemap