Urban road scene semantic segmentation method based on deep learning
A technology of semantic segmentation and deep learning, applied in neural learning methods, image analysis, details involving image mosaic, etc., to achieve the effect of less data sets, strong practicability and adaptability, and easy to understand
- Summary
- Abstract
- Description
- Claims
- Application Information
AI Technical Summary
Problems solved by technology
Method used
Image
Examples
Embodiment Construction
[0037] The method of the present invention will be described in further detail below in conjunction with the accompanying drawings.
[0038] refer to Figure 1 ~ Figure 4 , a method for semantic segmentation of urban road scenes based on deep learning, said method comprising the following steps:
[0039] 1) Image collection at the front end of the vehicle: regularly collect urban road images, set the time interval as T, and input the image with a resolution of h×w into the image detection module to obtain a valid image; then input the image into the labeling module to mark , the system uses the labeling software Labelme3.11.2 of the public image interface for labeling. Through its scene segmentation labeling function, the vehicles, pedestrians, bicycles, traffic lights and neon lights on the image are framed and labeled into different categories, and the generated labeling The image reflects different types of objects through different gray levels, and the gray table list and...
PUM
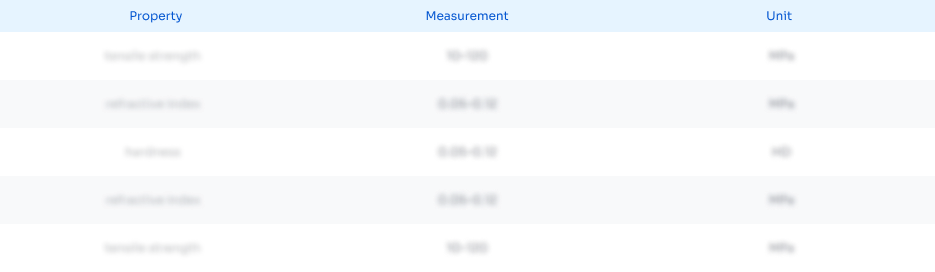
Abstract
Description
Claims
Application Information
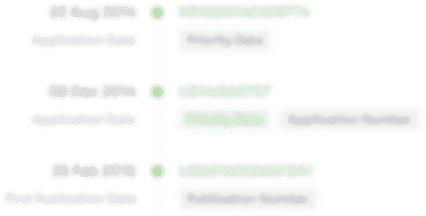
- R&D
- Intellectual Property
- Life Sciences
- Materials
- Tech Scout
- Unparalleled Data Quality
- Higher Quality Content
- 60% Fewer Hallucinations
Browse by: Latest US Patents, China's latest patents, Technical Efficacy Thesaurus, Application Domain, Technology Topic, Popular Technical Reports.
© 2025 PatSnap. All rights reserved.Legal|Privacy policy|Modern Slavery Act Transparency Statement|Sitemap|About US| Contact US: help@patsnap.com