Expression recognition method based on multi-branch cross-connection convolutional neural network
A technology of convolutional neural network and expression recognition, which is applied in the field of expression recognition based on multi-branch cross-connection convolutional neural network, can solve the problems of incomplete feature extraction, waste of resources, and low efficiency, so as to improve the ability of feature extraction and improve Performance, Effects of Effective Image Feature Extraction
- Summary
- Abstract
- Description
- Claims
- Application Information
AI Technical Summary
Problems solved by technology
Method used
Image
Examples
specific Embodiment approach 1
[0015] Specific implementation mode 1: The specific process of the facial expression recognition method based on multi-branch cross-connection convolutional neural network in this implementation mode is as follows:
[0016] Expression recognition is a classic research topic in the field of computer vision. Existing expression recognition methods can be roughly divided into three categories: expression recognition based on traditional methods, expression recognition based on convolutional neural networks, and expression recognition methods based on fusion of traditional methods and convolutional neural networks.
[0017] For expression recognition based on traditional methods, handcrafted features such as Gabor wavelet coefficients [16] (TianYL, Cohn J F.Evaluation of Gabor-Wavelet-Based Facial Action Unit Recognition in Image Sequences of Increasing Complexity[C] / / Automatic Face and GestureRecognition,2002.Proceedings.Fifth IEEE International Conference on.IEEE,2002.), local ...
specific Embodiment approach 2
[0027] Specific embodiment two: the difference between this embodiment and specific embodiment one is: in the described step one, the facial expression image data set is preprocessed; the specific process is:
[0028] Select Fer2013 and CK+ facial expression data sets, normalize the facial expression data sets, and perform data enhancement on the normalized data;
[0029] The process of data augmentation on the normalized data is as follows:
[0030] Randomly zoom, flip, translate, and rotate the normalized data;
[0031] Other steps and parameters are the same as those in Embodiment 1.
specific Embodiment approach 3
[0032] Specific embodiment three: what this embodiment is different from specific embodiment one or two is: build multi-branch cross-connection convolutional neural network (MBCC-CNN) in described step 2, be used to extract facial expression image feature; Concrete process for:
[0033] The multi-branch cross-connection convolutional neural network consists of the first convolutional layer, module 1, module 2 and module 3, the fortieth convolutional layer, batch normalization BN (BatchNormalization) and Relu activation function;
[0034] Module 1 includes a second convolutional layer, a third convolutional layer, and a fourth convolutional layer, a twenty-first convolutional layer, a twenty-second convolutional layer, and a twenty-third convolutional layer;
[0035] The image data of the face dataset is the input layer, the input layer data is input to the first convolutional layer, the output data of the first convolutional layer is respectively input into the second convolut...
PUM
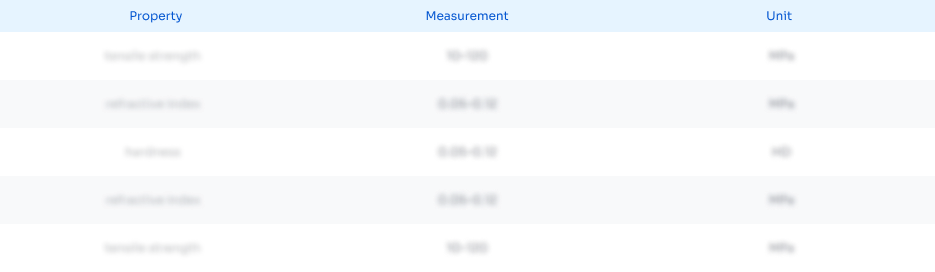
Abstract
Description
Claims
Application Information
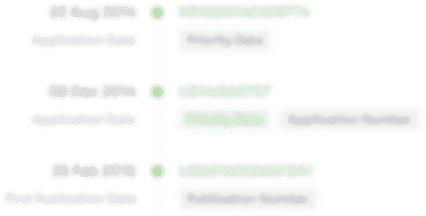
- R&D Engineer
- R&D Manager
- IP Professional
- Industry Leading Data Capabilities
- Powerful AI technology
- Patent DNA Extraction
Browse by: Latest US Patents, China's latest patents, Technical Efficacy Thesaurus, Application Domain, Technology Topic, Popular Technical Reports.
© 2024 PatSnap. All rights reserved.Legal|Privacy policy|Modern Slavery Act Transparency Statement|Sitemap|About US| Contact US: help@patsnap.com