Disease prognosis prediction system based on deep semi-supervised multi-task learning survival analysis
A multi-task learning and survival analysis technology, applied in the field of disease prognosis prediction system, can solve problems such as censored data, inability to make full use of censored data, and inability to provide complete information from the starting point to the event, etc.
- Summary
- Abstract
- Description
- Claims
- Application Information
AI Technical Summary
Problems solved by technology
Method used
Image
Examples
Embodiment Construction
[0073] In order to make the above objects, features and advantages of the present invention more comprehensible, specific implementations of the present invention will be described in detail below in conjunction with the accompanying drawings.
[0074] In the following description, a lot of specific details are set forth in order to fully understand the present invention, but the present invention can also be implemented in other ways different from those described here, and those skilled in the art can do it without departing from the meaning of the present invention. By analogy, the present invention is therefore not limited to the specific examples disclosed below.
[0075] The censored data in this application is: if there is no result event at the specified end time, it is called censored data, and the time from the start point to censored is called censored time. The time-dependent phenomenon is: regardless of the baseline risk, at any point in time, the risk of an event...
PUM
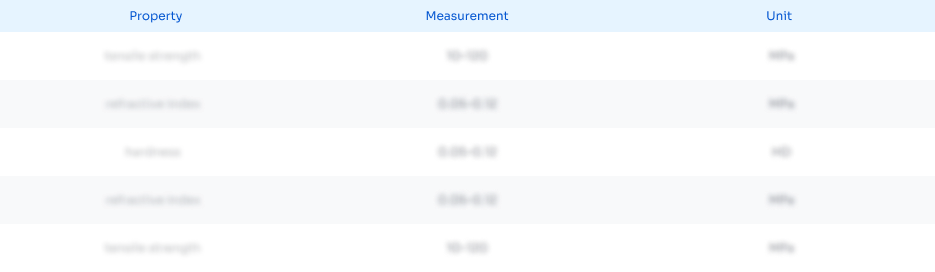
Abstract
Description
Claims
Application Information
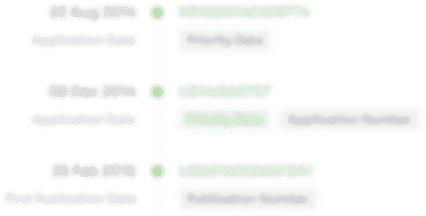
- R&D Engineer
- R&D Manager
- IP Professional
- Industry Leading Data Capabilities
- Powerful AI technology
- Patent DNA Extraction
Browse by: Latest US Patents, China's latest patents, Technical Efficacy Thesaurus, Application Domain, Technology Topic.
© 2024 PatSnap. All rights reserved.Legal|Privacy policy|Modern Slavery Act Transparency Statement|Sitemap