Multi-scale target detection model method based on metric learning
A technique of metric learning and target detection, applied in neural learning methods, biological neural network models, character and pattern recognition, etc.
- Summary
- Abstract
- Description
- Claims
- Application Information
AI Technical Summary
Problems solved by technology
Method used
Image
Examples
Embodiment Construction
[0084] Below in conjunction with accompanying drawing, further describe the present invention through embodiment, but do not limit the scope of the present invention in any way.
[0085] Method flow chart as figure 1 As shown, the model structure is as figure 2 shown. The method of the present invention includes: 1) building a multi-scale target detection model based on metric learning: the model adopts a two-step detection strategy to realize the detection and recognition of the target, a region of interest extraction module, and a target classification and recognition module; 2) pre-training multiple Scale model: Train the border regression prediction of the multi-scale model part of the model on a large-scale data set, and then transfer learning to this model for fine-tuning to extract the region of interest in the image; 3) Metric learning target detection: use The fully connected layer learns the convolution features, and identifies the region of interest by measuring ...
PUM
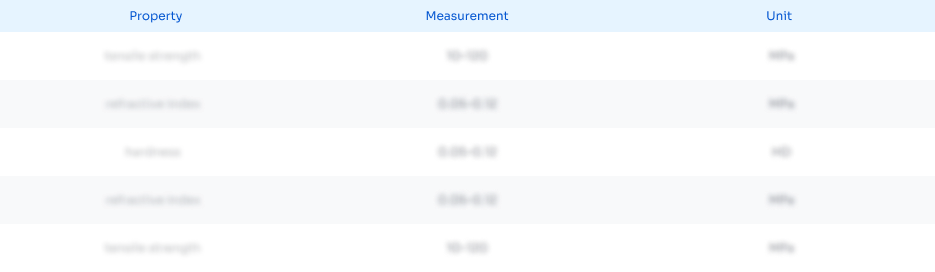
Abstract
Description
Claims
Application Information
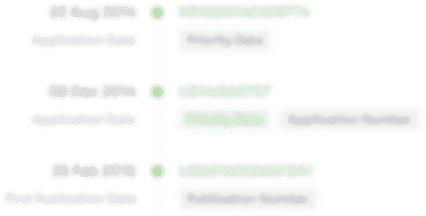
- R&D Engineer
- R&D Manager
- IP Professional
- Industry Leading Data Capabilities
- Powerful AI technology
- Patent DNA Extraction
Browse by: Latest US Patents, China's latest patents, Technical Efficacy Thesaurus, Application Domain, Technology Topic, Popular Technical Reports.
© 2024 PatSnap. All rights reserved.Legal|Privacy policy|Modern Slavery Act Transparency Statement|Sitemap|About US| Contact US: help@patsnap.com