Super-dense edge computing network mobility management method based on deep reinforcement learning
A technology of reinforcement learning and edge computing, applied in neural learning methods, network traffic/resource management, based on specific mathematical models, etc.
- Summary
- Abstract
- Description
- Claims
- Application Information
AI Technical Summary
Problems solved by technology
Method used
Image
Examples
Embodiment Construction
[0094] The present invention provides a mobility management method for ultra-dense edge computing networks based on deep reinforcement learning, which can be well implemented in the case of dense deployment of wireless access nodes and edge computing nodes. In the process, mobility management decisions are provided for multiple wireless access nodes and multiple edge computing nodes. In addition, simply considering the performance of nodes to select the optimal performance decision will lead to the problem of frequent migration. Therefore, the present invention studies the balance between user quality of service (QoS) and service mobility in the process of user mobility, that is, in the user During the mobile process, select the appropriate wireless access point and edge server to ensure the user's QoS while reducing the service migration rate at a certain rate. The present invention regards the processing delay of computing tasks as the index of user QoS, and proposes a metho...
PUM
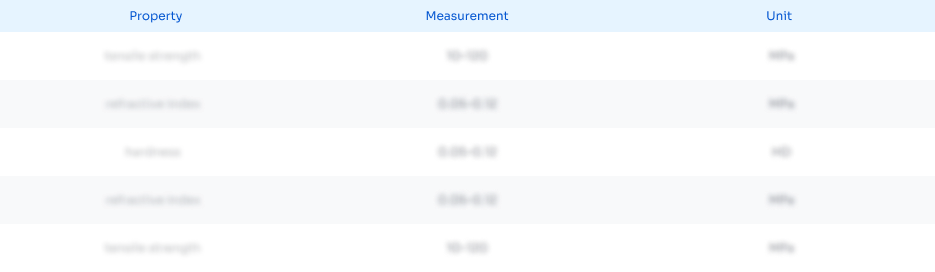
Abstract
Description
Claims
Application Information
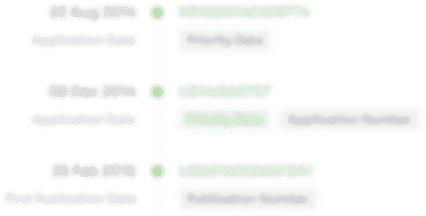
- R&D
- Intellectual Property
- Life Sciences
- Materials
- Tech Scout
- Unparalleled Data Quality
- Higher Quality Content
- 60% Fewer Hallucinations
Browse by: Latest US Patents, China's latest patents, Technical Efficacy Thesaurus, Application Domain, Technology Topic, Popular Technical Reports.
© 2025 PatSnap. All rights reserved.Legal|Privacy policy|Modern Slavery Act Transparency Statement|Sitemap|About US| Contact US: help@patsnap.com