Seismic phase feature recognition waveform inversion method based on full convolutional neural network
A technology of convolutional neural network and waveform inversion, which is applied in the field of exploration seismic waveform recognition technology and deep learning, can solve the problems of slow convergence, non-convergence, waveform window accuracy and efficiency of inversion, etc., to improve convergence, The effect of improving inversion efficiency
- Summary
- Abstract
- Description
- Claims
- Application Information
AI Technical Summary
Problems solved by technology
Method used
Image
Examples
Embodiment Construction
[0036] Below in conjunction with accompanying drawing and specific embodiment, further illustrate the present invention, should be understood that these examples are only for illustrating the present invention and are not intended to limit the scope of the present invention, after having read the present invention, those skilled in the art will understand various aspects of the present invention All modifications of the valence form fall within the scope defined by the appended claims of the present application.
[0037] A waveform inversion method for phase feature recognition based on fully convolutional neural network, such as figure 1 As shown in Fig. 1, a full convolutional neural network window selection mechanism is added to the traditional full waveform inversion process to avoid cycle jumps, including the following steps:
[0038] Step 1. Obtain the actual observed seismic records as observed waveform data. Obtain earthquake training data samples, and use the traditi...
PUM
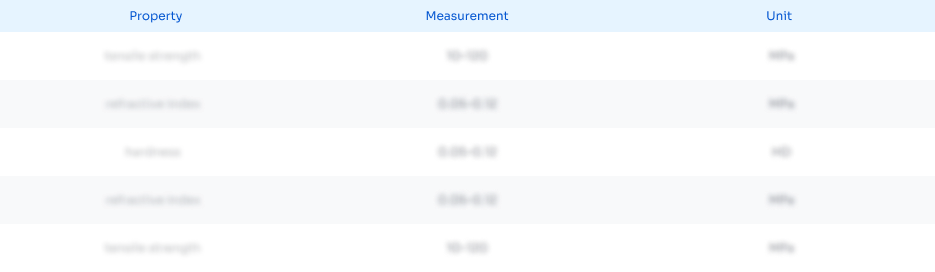
Abstract
Description
Claims
Application Information
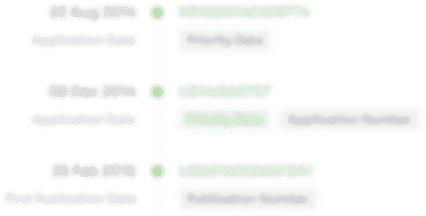
- R&D Engineer
- R&D Manager
- IP Professional
- Industry Leading Data Capabilities
- Powerful AI technology
- Patent DNA Extraction
Browse by: Latest US Patents, China's latest patents, Technical Efficacy Thesaurus, Application Domain, Technology Topic, Popular Technical Reports.
© 2024 PatSnap. All rights reserved.Legal|Privacy policy|Modern Slavery Act Transparency Statement|Sitemap|About US| Contact US: help@patsnap.com