Robust training method for target detection network
A technology of target detection and training method, which is applied in the field of robust training for target detection network to achieve the effect of improving anti-interference
- Summary
- Abstract
- Description
- Claims
- Application Information
AI Technical Summary
Problems solved by technology
Method used
Image
Examples
Embodiment Construction
[0012] Hereinafter, exemplary embodiments of the present application will be described in detail with reference to the accompanying drawings. Apparently, the described embodiments are only some of the embodiments of the present application, rather than all the embodiments of the present application. It should be understood that the present application is not limited by the exemplary embodiments described here.
[0013] Application overview
[0014] Taking FasterRCNN in the target detection network as an example, FasterRCNN will generate a suggestion frame during training, and then calculate the intersection ratio between the suggestion frame and the label frame. If the intersection ratio is greater than the manually set threshold, the suggestion frame will be marked with a category label ( Positive sample), otherwise label the background (negative sample), and use this label as a positive and negative sample to train the network. However, if the artificial annotation box in t...
PUM
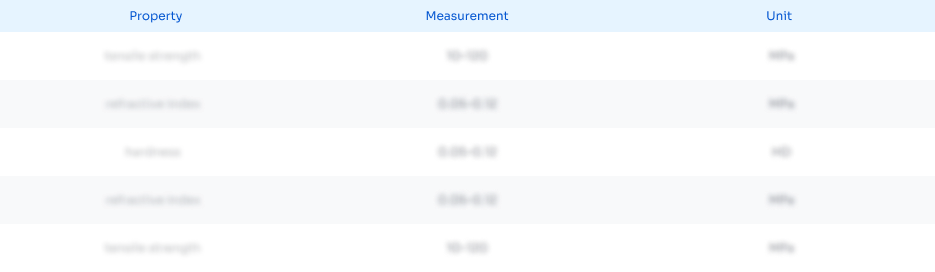
Abstract
Description
Claims
Application Information
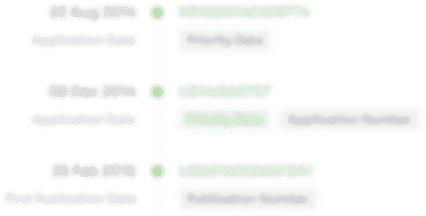
- R&D
- Intellectual Property
- Life Sciences
- Materials
- Tech Scout
- Unparalleled Data Quality
- Higher Quality Content
- 60% Fewer Hallucinations
Browse by: Latest US Patents, China's latest patents, Technical Efficacy Thesaurus, Application Domain, Technology Topic, Popular Technical Reports.
© 2025 PatSnap. All rights reserved.Legal|Privacy policy|Modern Slavery Act Transparency Statement|Sitemap|About US| Contact US: help@patsnap.com