Image classification method based on adversarial fusion multi-source transfer learning
A technology of transfer learning and classification methods, which is applied in the field of image classification and image classification under the absence of training data labels, which can solve the problems of source domain data losing effective features and affecting classification effects, so as to improve classification accuracy and classification accuracy Effect
- Summary
- Abstract
- Description
- Claims
- Application Information
AI Technical Summary
Problems solved by technology
Method used
Image
Examples
Embodiment Construction
[0031] The embodiments and effects of the present invention will be further described in detail below in conjunction with the accompanying drawings.
[0032] refer to figure 1 , where S 1 ... S N Represents N source domains, T represents the target domain, F and F 1 ...F N Denote domain-shared subnetworks and N domain-specific subnetworks respectively, D 1 ...D N Denotes N domain discriminators, C 1 ...C N Represents N classifiers, and the specific implementation steps are as follows:
[0033] Step 1. Establish a domain-shared subnetwork F and a domain-specific subnetwork F j Constituted feature extraction network.
[0034] The domain sharing subnetwork F is a residual neural network ResNet50 proposed by He Yuming et al. The network consists of a convolutional layer followed by 4 residual blocks, aiming to extract the underlying features shared by all domains;
[0035]There are a total of N domain-specific sub-networks. Each sub-network is a multi-layer neural networ...
PUM
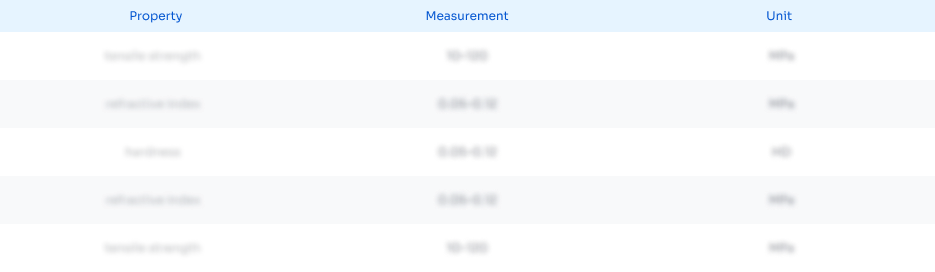
Abstract
Description
Claims
Application Information
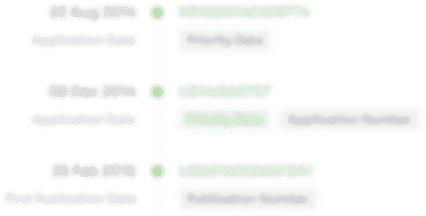
- R&D
- Intellectual Property
- Life Sciences
- Materials
- Tech Scout
- Unparalleled Data Quality
- Higher Quality Content
- 60% Fewer Hallucinations
Browse by: Latest US Patents, China's latest patents, Technical Efficacy Thesaurus, Application Domain, Technology Topic, Popular Technical Reports.
© 2025 PatSnap. All rights reserved.Legal|Privacy policy|Modern Slavery Act Transparency Statement|Sitemap|About US| Contact US: help@patsnap.com