Coflow scheduling method based on deep reinforcement learning
A technology of reinforcement learning and scheduling methods, applied in biological neural network models, digital transmission systems, electrical components, etc., can solve problems such as limiting the final effect of scheduling, suboptimal thresholds, complex network environments, etc., to achieve flexible scheduling strategies, Reduce overhead and improve processing efficiency
- Summary
- Abstract
- Description
- Claims
- Application Information
AI Technical Summary
Problems solved by technology
Method used
Image
Examples
Embodiment Construction
[0047] The technical solutions in the embodiments of the present invention will be clearly and completely described below in conjunction with the accompanying drawings in the embodiments of the present invention. Obviously, the described embodiments are only part of the embodiments of the present invention, not all of them. Based on the embodiments of the present invention, all other embodiments obtained by persons of ordinary skill in the art without making creative efforts belong to the protection scope of the present invention.
[0048] The present invention is based on the working principle of deep reinforcement learning. Among them, reinforcement learning is an important branch of machine learning. In reinforcement learning, there is an agent for interacting with the environment. The agent takes corresponding actions by observing the state of the environment to obtain the maximum reward value feedback. Reinforcement learning is usually formulated using a Markov decision p...
PUM
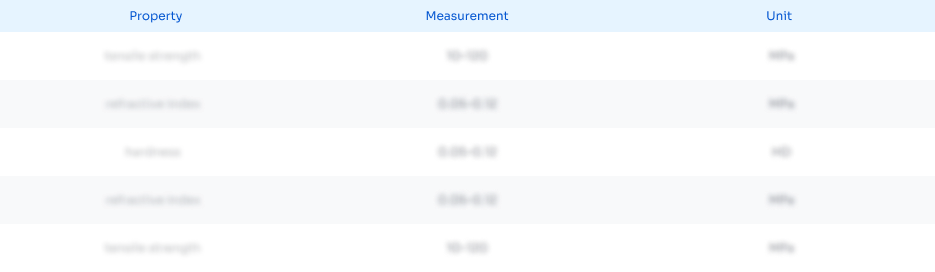
Abstract
Description
Claims
Application Information
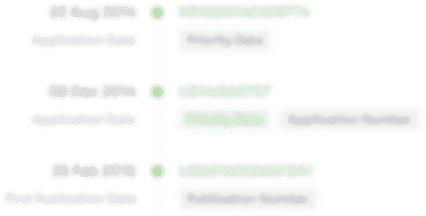
- R&D Engineer
- R&D Manager
- IP Professional
- Industry Leading Data Capabilities
- Powerful AI technology
- Patent DNA Extraction
Browse by: Latest US Patents, China's latest patents, Technical Efficacy Thesaurus, Application Domain, Technology Topic, Popular Technical Reports.
© 2024 PatSnap. All rights reserved.Legal|Privacy policy|Modern Slavery Act Transparency Statement|Sitemap|About US| Contact US: help@patsnap.com