Method for correcting frequency response dynamic model of a few-sample structure based on transfer learning
A technology of transfer learning and model correction, applied in biological neural network models, neural architecture, design optimization/simulation, etc., to improve the accuracy of model correction, solve the problem of network overfitting and accuracy decline, and avoid errors.
- Summary
- Abstract
- Description
- Claims
- Application Information
AI Technical Summary
Problems solved by technology
Method used
Image
Examples
Embodiment Construction
[0026] The invention provides a method for correcting the frequency response dynamics model of a few-sample structure based on migration learning.
[0027] The calculation example of the present invention adopts a certain aircraft structure, and its finite element model is shown in image 3 , see the simulation results Figure 4 . The numerical example of the present invention selects 6 parameters to be corrected: the elastic modulus θ of the main structure 1 , the density θ of the main structure 2 , the thickness of the central cylinder (central cylinder) θ 3 , thickness of lower platform θ 4 , the thickness of shear panels θ 5 and the thickness of the upper platform θ 6 . , the parameters to be corrected and their real values are shown in Table 1.
[0028] Step 1: Set the number of samples to 100-10000 for comparison and verification, then generate the initial distribution range of the parameters to be corrected according to the actual working conditions, and recor...
PUM
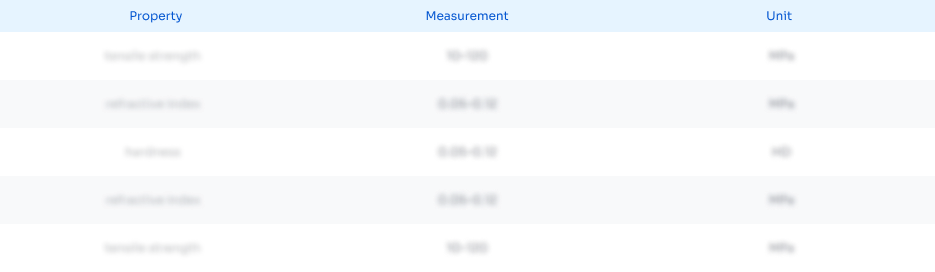
Abstract
Description
Claims
Application Information
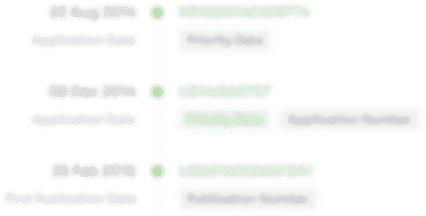
- R&D Engineer
- R&D Manager
- IP Professional
- Industry Leading Data Capabilities
- Powerful AI technology
- Patent DNA Extraction
Browse by: Latest US Patents, China's latest patents, Technical Efficacy Thesaurus, Application Domain, Technology Topic, Popular Technical Reports.
© 2024 PatSnap. All rights reserved.Legal|Privacy policy|Modern Slavery Act Transparency Statement|Sitemap|About US| Contact US: help@patsnap.com