Semantic vision positioning method and device based on multi-modal graph convolutional network
A convolutional network, multi-modal technology, applied in the computer field, can solve the problems of lack of semantic relationship information exploration, limited, difficult to obtain visual positioning, etc., to achieve the effect of improving task performance, alleviating the impact, and obtaining accurate acquisition.
- Summary
- Abstract
- Description
- Claims
- Application Information
AI Technical Summary
Problems solved by technology
Method used
Image
Examples
Embodiment Construction
[0024] Exemplary embodiments of the present disclosure will be described in more detail below with reference to the accompanying drawings. Although exemplary embodiments of the present disclosure are shown in the drawings, it should be understood that the present disclosure may be embodied in various forms and should not be limited by the embodiments set forth herein. Rather, these embodiments are provided for more thorough understanding of the present disclosure and to fully convey the scope of the present disclosure to those skilled in the art.
[0025] The core of the present invention lies in: proposing to construct a semantic structure graph by parsing corpus, learning and extracting multi-modal features under the guidance of semantic information, and improving the performance of semantic visual positioning tasks. This method constructs a semantic structure graph based on semantic information by parsing corpus input, uses multimodal features that combine visual features, ...
PUM
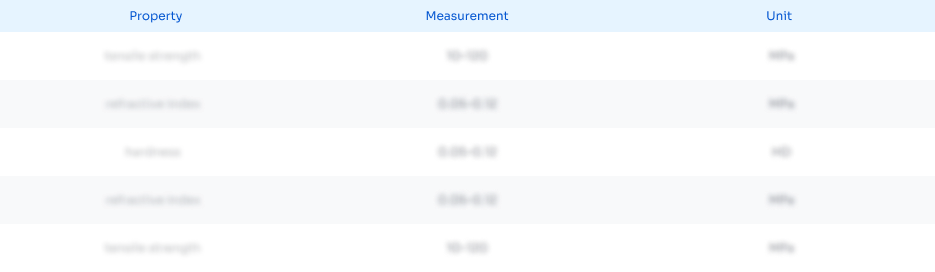
Abstract
Description
Claims
Application Information
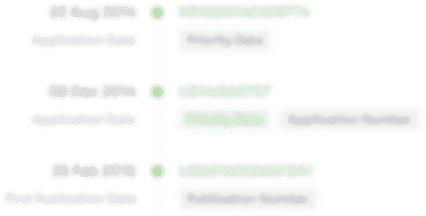
- R&D Engineer
- R&D Manager
- IP Professional
- Industry Leading Data Capabilities
- Powerful AI technology
- Patent DNA Extraction
Browse by: Latest US Patents, China's latest patents, Technical Efficacy Thesaurus, Application Domain, Technology Topic, Popular Technical Reports.
© 2024 PatSnap. All rights reserved.Legal|Privacy policy|Modern Slavery Act Transparency Statement|Sitemap|About US| Contact US: help@patsnap.com