Adversarial sample defense method based on Bayesian convolutional neural network
A convolutional neural network and adversarial sample technology, applied in the direction of biological neural network models, neural architectures, instruments, etc., can solve the problem of not being robust, unable to have robust image recognition systems, unable to achieve accurate image recognition and classification, etc. problem, to achieve the effect of improving robustness and image recognition accuracy
- Summary
- Abstract
- Description
- Claims
- Application Information
AI Technical Summary
Problems solved by technology
Method used
Image
Examples
Embodiment Construction
[0029] In order to make the purpose, technical solution and advantages of the present invention clearer, the technical solution of the present invention will be clearly and completely described below in conjunction with specific embodiments of the present invention and corresponding drawings. Apparently, the described embodiments are only some of the embodiments of the present invention, but not all of them. Based on the embodiments of the present invention, all other embodiments obtained by persons of ordinary skill in the art without making creative efforts fall within the protection scope of the present invention.
[0030] The technical solutions provided by the embodiments of the present invention will be described in detail below in conjunction with the accompanying drawings.
[0031] as attached figure 1 As shown, an embodiment of the present invention provides a method for defending against samples based on Bayesian convolutional neural network, the method is used to t...
PUM
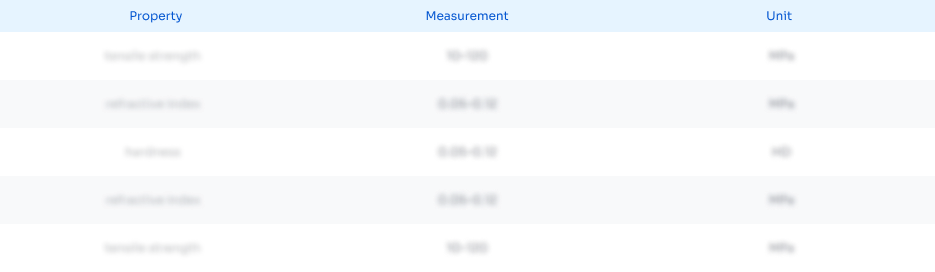
Abstract
Description
Claims
Application Information
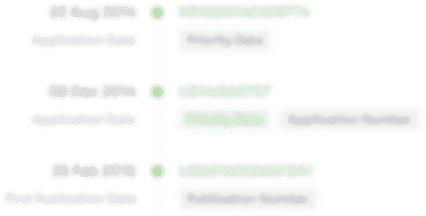
- R&D Engineer
- R&D Manager
- IP Professional
- Industry Leading Data Capabilities
- Powerful AI technology
- Patent DNA Extraction
Browse by: Latest US Patents, China's latest patents, Technical Efficacy Thesaurus, Application Domain, Technology Topic, Popular Technical Reports.
© 2024 PatSnap. All rights reserved.Legal|Privacy policy|Modern Slavery Act Transparency Statement|Sitemap|About US| Contact US: help@patsnap.com