Method for seven-day prediction of 24-point power load values based on optimized LSTM network
A technology of power load and forecasting method, which is applied in the field of 7-day forecasting of 24-point power load value based on optimized LSTM network, which can solve problems such as difficult forecasting, large dimension differences, and complex relationships, so as to reduce the proportion of influence and improve accuracy and time-sensitive effects
- Summary
- Abstract
- Description
- Claims
- Application Information
AI Technical Summary
Problems solved by technology
Method used
Image
Examples
Embodiment Construction
[0058] The technical solutions in the present invention will be further described below in conjunction with the accompanying drawings and embodiments.
[0059] Such as figure 1 As shown, the present invention proposes a 24-point power load value 7-day prediction method based on an optimized LSTM network, comprising the following steps;
[0060] Step S1. Acquire the historical power load data set. The experimental data comes from the power load data of the 1# main transformer of Aoshan Substation of Chongqing Power Grid. The sampling interval of the data set is 60 minutes, that is, the load value at 24 points per day. The data set contains more than 3 years of load data from November 27, 2014 to December 31, 2017, with a total of 27,144 items.
[0061] Step S2, data analysis: the acquired power load data has a strong time correlation, showing a stable periodic change. By drawing the power load curve, some universal characteristics of the power load data are obtained, including...
PUM
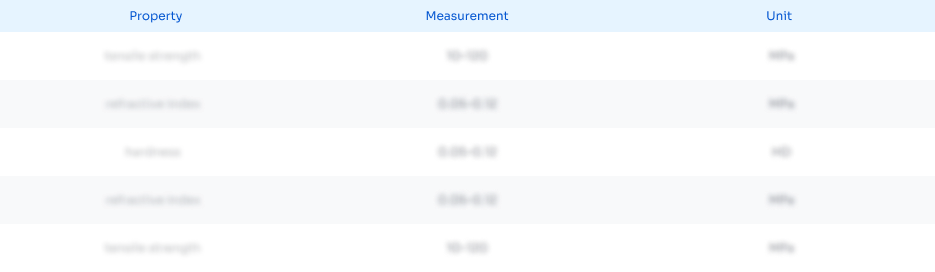
Abstract
Description
Claims
Application Information
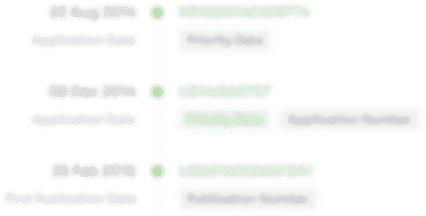
- R&D Engineer
- R&D Manager
- IP Professional
- Industry Leading Data Capabilities
- Powerful AI technology
- Patent DNA Extraction
Browse by: Latest US Patents, China's latest patents, Technical Efficacy Thesaurus, Application Domain, Technology Topic, Popular Technical Reports.
© 2024 PatSnap. All rights reserved.Legal|Privacy policy|Modern Slavery Act Transparency Statement|Sitemap|About US| Contact US: help@patsnap.com