Deep learning model suitable for small sample hyperspectral image classification
An image classification and deep learning technology, applied in the field of deep learning and remote sensing image processing, can solve problems such as manual, inability to achieve end-to-end training, achieve good classification accuracy, solve the problem of spectral information redundancy, and improve performance.
- Summary
- Abstract
- Description
- Claims
- Application Information
AI Technical Summary
Problems solved by technology
Method used
Image
Examples
Embodiment Construction
[0052] In order to have a clearer understanding of the technical solutions, objectives and effects of the present invention, the specific implementation manners of the present invention will now be described in detail with reference to the accompanying drawings.
[0053] DETAILED DESCRIPTION A deep learning model suitable for small sample hyperspectral image classification is disclosed, such as figure 1 As shown, the specific operation steps are as follows:
[0054] S1. Input the hyperspectral images to be classified, and input the sample data sets corresponding to the hyperspectral images to be classified; this embodiment uses a total of three sets of hyperspectral images, but in this example only the IndianPines hyperspectral images and data set for analysis and discussion. The surface coverage of the images is mainly agricultural planting areas, with a spatial resolution of 20m and a wavelength range of 0.4-2.5μm. There are a total of 220 bands. After removing the water ab...
PUM
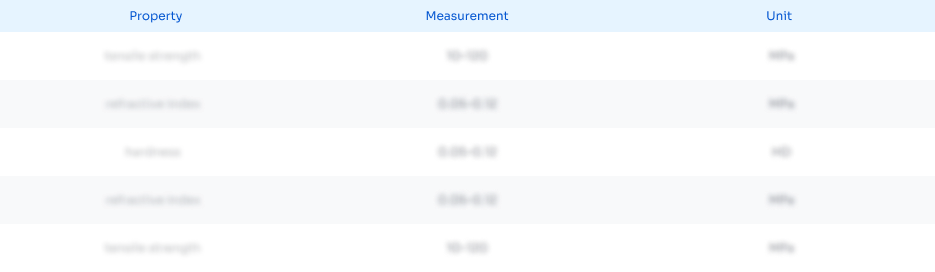
Abstract
Description
Claims
Application Information
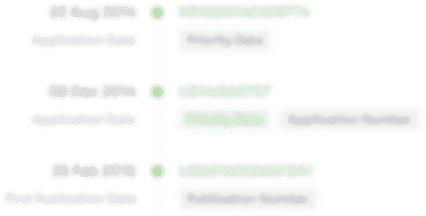
- R&D Engineer
- R&D Manager
- IP Professional
- Industry Leading Data Capabilities
- Powerful AI technology
- Patent DNA Extraction
Browse by: Latest US Patents, China's latest patents, Technical Efficacy Thesaurus, Application Domain, Technology Topic, Popular Technical Reports.
© 2024 PatSnap. All rights reserved.Legal|Privacy policy|Modern Slavery Act Transparency Statement|Sitemap|About US| Contact US: help@patsnap.com