Robust vision SLAM method based on semantic prior and deep learning features
A deep learning and semantic technology, applied in character and pattern recognition, instruments, manipulators, etc., can solve problems such as insufficient indoor navigation, reducing the accuracy of visual SLAM algorithm's own pose estimation, and visual SLAM framework not considering the impact of dynamic objects. , to achieve the effect of simple principle
- Summary
- Abstract
- Description
- Claims
- Application Information
AI Technical Summary
Problems solved by technology
Method used
Image
Examples
Embodiment Construction
[0031] Such as figure 1 Shown, the concrete realization steps of the present invention are as follows:
[0032] Step 1. Build a visual SLAM framework based on deep learning feature extraction, and initially achieve more robust visual positioning performance in weak texture and dynamic scenes.
[0033] The current classic visual SLAM framework, the extracted features are all artificially designed features, and the ORB-SLAM framework is the main one, and the ORB features are extracted. With the continuous development of deep learning, feature extraction methods based on deep learning have received extensive attention. The image features extracted by deep learning can express image information more fully and have stronger robustness to environmental changes such as illumination. In addition, the feature extraction method based on deep learning can obtain multi-level image features, combining low-level features (such as pixel-level grayscale features) and high-level features (su...
PUM
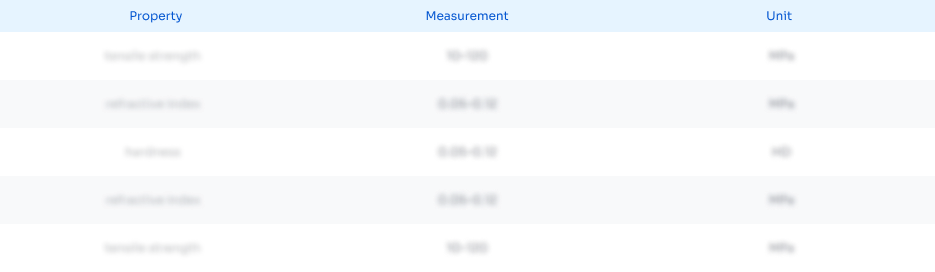
Abstract
Description
Claims
Application Information
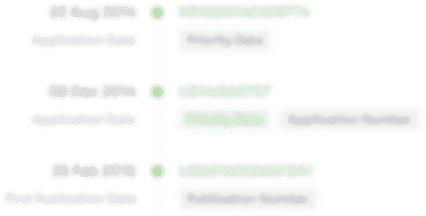
- R&D Engineer
- R&D Manager
- IP Professional
- Industry Leading Data Capabilities
- Powerful AI technology
- Patent DNA Extraction
Browse by: Latest US Patents, China's latest patents, Technical Efficacy Thesaurus, Application Domain, Technology Topic, Popular Technical Reports.
© 2024 PatSnap. All rights reserved.Legal|Privacy policy|Modern Slavery Act Transparency Statement|Sitemap|About US| Contact US: help@patsnap.com