Frequency hopping signal detection and parameter estimation method based on deep neural network
A deep neural network and frequency hopping signal technology, applied in the field of frequency hopping signal detection and parameter estimation
- Summary
- Abstract
- Description
- Claims
- Application Information
AI Technical Summary
Problems solved by technology
Method used
Image
Examples
Embodiment Construction
[0028] The technical solutions involved in the present invention will be further described in detail below in conjunction with the accompanying drawings and specific implementation examples. It should be understood that the preferred embodiments described below are only used to illustrate and explain the present invention, and are not intended to limit the present invention:
[0029] 1. Generation and collection of frequency hopping signal data
[0030] Object detection requires a large amount of labeled data for training and learning. For frequency hopping signals, it is difficult to collect a large amount of real data of frequency hopping signals that meet the training requirements in a short period of time. Therefore the present invention emulates and generates 3 kinds of digital modulation modes (BPSK, QPSK, QAM); The sampling rate is 3.2MHz; MHz, 2.56MHz}, {2.88MHz, 2.24MHz, 1.6MHz, 0.96MHz}, {2.56MHz, 1.92MHz, 1.28MHz, 0.64MHz} frequency hopping signals with a signal-to...
PUM
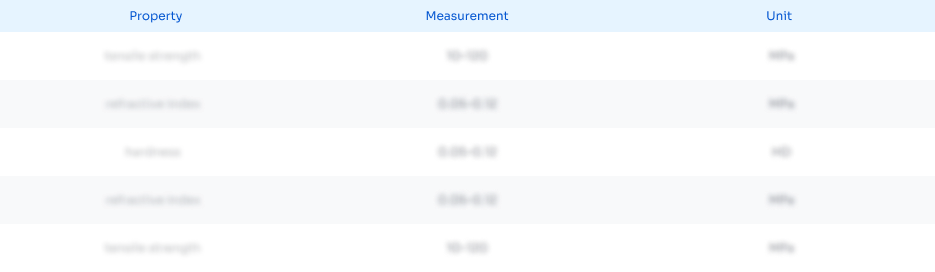
Abstract
Description
Claims
Application Information
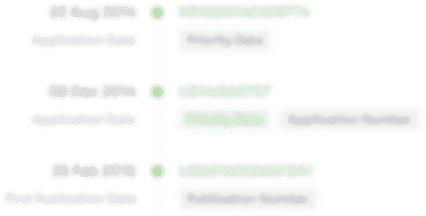
- R&D Engineer
- R&D Manager
- IP Professional
- Industry Leading Data Capabilities
- Powerful AI technology
- Patent DNA Extraction
Browse by: Latest US Patents, China's latest patents, Technical Efficacy Thesaurus, Application Domain, Technology Topic, Popular Technical Reports.
© 2024 PatSnap. All rights reserved.Legal|Privacy policy|Modern Slavery Act Transparency Statement|Sitemap|About US| Contact US: help@patsnap.com