Knowledge graph representation learning method based on ternary interaction
A knowledge graph and learning method technology, applied in special data processing applications, unstructured text data retrieval, instruments, etc., can solve problems such as time complexity obstacles, achieve strong universality, high practical value, and reduce dependence. Effect
- Summary
- Abstract
- Description
- Claims
- Application Information
AI Technical Summary
Problems solved by technology
Method used
Image
Examples
Embodiment 1
[0094] Embodiment 1. Link Prediction
[0095] Link prediction is one of the common experiments to verify the effectiveness of knowledge graph representation learning results. Its task is to predict the third based on two of head entity, relation and tail entity, specifically including head entity prediction, tail entity prediction and relation prediction. Same as the existing embedding knowledge graph representation learning model, this embodiment also adopts MeanRank and Hit@k two indicators, and reports raw (before filtering) and filt (after filtering) results. Among them, all k=10, ie Hit@10, except that the relation of ES is predicted to be Hit@1.
[0096] Based on AdaGrad, the learning rate of this embodiment is 0.1, and the maximum number of training rounds is 1000. The tuning range is the learning rate attenuation coefficient γ∈{0.1,0.01,0.001}, and the number of negative examples n∈{3,4,5,6}. Through grid search, this embodiment obtains that the optimal parameters o...
Embodiment 2
[0109] Embodiment 2, triple group classification
[0110] In knowledge graph representation learning, triple classification is also a common experiment to test the effectiveness of the model. The task of this experiment is to judge whether a given triplet holds or not. The experimental details of this embodiment are consistent with the traditional experiments. The baseline and parameter adjustment method of triplet classification are consistent with the link prediction above. Based on the grid search, the optimal parameters obtained in this embodiment are: ES data set γ=0.001, n=3; Kinship data set γ=0.01, n=5.
[0111] Table 4 is the experimental results for triplet classification. Whether it is the ES dataset or the Kinship dataset, the accuracy of the InterTris model is the highest. Especially for the Kinship dataset, the accuracy of the InterTris model is 17.69 percentage points higher than that of the second-ranked TranSparse.
[0112] Table 4 Triad classification ex...
PUM
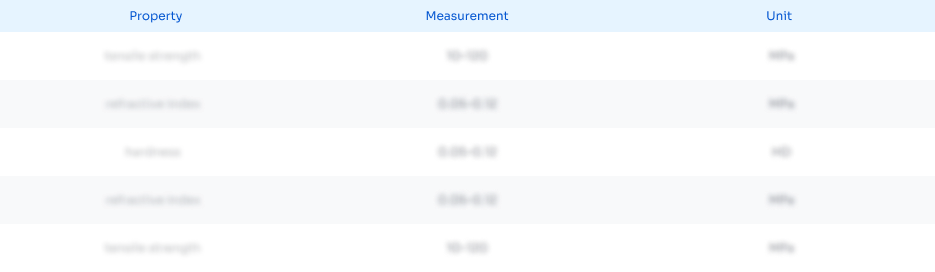
Abstract
Description
Claims
Application Information
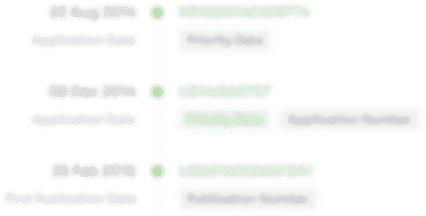
- R&D Engineer
- R&D Manager
- IP Professional
- Industry Leading Data Capabilities
- Powerful AI technology
- Patent DNA Extraction
Browse by: Latest US Patents, China's latest patents, Technical Efficacy Thesaurus, Application Domain, Technology Topic, Popular Technical Reports.
© 2024 PatSnap. All rights reserved.Legal|Privacy policy|Modern Slavery Act Transparency Statement|Sitemap|About US| Contact US: help@patsnap.com