Two-channel exposure fusion network model and method for low-light image enhancement
An image enhancement and fusion network technology, applied in image enhancement, biological neural network model, image analysis, etc., can solve the problems of unnatural enhancement results, limited generalization ability, oversaturation, etc., and achieve good noise suppression effect
- Summary
- Abstract
- Description
- Claims
- Application Information
AI Technical Summary
Problems solved by technology
Method used
Image
Examples
no. 1 example
[0046] In order to solve the problems existing in the existing methods, this embodiment proposes a dual exposure fusion structure model for low-light image enhancement based on a convolutional neural network. The network model is inspired by the imitation of the human creative process, that is, good works can obtain empirical guidance (fusion) from multiple attempts (generation). This embodiment considers that this generation and fusion strategy can also be adopted for the enhancement of low-illumination images. Since brightness is one of the most important and most easily changed factors in the imaging process, the enhancement strategy in this embodiment is positioned on how to perform desired enhancement for images with various unknown illumination levels.
[0047] For this reason, in the image generation stage, the method proposed in this embodiment uses a two-branch structure, and uses different enhancement strategies to deal with the extremely low and low illumination con...
no. 2 example
[0177] This embodiment provides a dual exposure fusion method for low-illumination image enhancement, which can be implemented by an electronic device. The execution flow of this method is as follows Figure 4 shown, including the following steps:
[0178] S101, processing the low-illumination image to be enhanced using different preset enhancement strategies, so as to obtain a two-way enhancement result corresponding to the low-illumination image to be enhanced;
[0179] S102, performing weighted fusion of the two-way enhancement results corresponding to the low-illumination images to be enhanced obtained by adopting different enhancement strategies, so as to obtain enhanced images corresponding to the low-illumination images to be enhanced;
[0180] Wherein, in the above S101, the image to be enhanced is enhanced using the following formula:
[0181]
[0182] in, represents the enhanced image, represents the input image to be enhanced, represents element-wise dot ...
no. 3 example
[0191] This embodiment provides an electronic device, which includes a processor and a memory; wherein, at least one instruction is stored in the memory, and the instruction is loaded and executed by the processor to implement the method of the second embodiment.
[0192] The electronic device may vary greatly due to different configurations or performances, and may include one or more processors (central processing units, CPU) and one or more memories, wherein the memory stores at least one instruction, so The instructions described above are loaded by the processor and perform the following steps:
[0193] S101, processing the low-illumination image to be enhanced using different preset enhancement strategies, so as to obtain a two-way enhancement result corresponding to the low-illumination image to be enhanced;
[0194] S102, performing weighted fusion of the two-way enhancement results corresponding to the low-illumination images to be enhanced obtained by adopting differ...
PUM
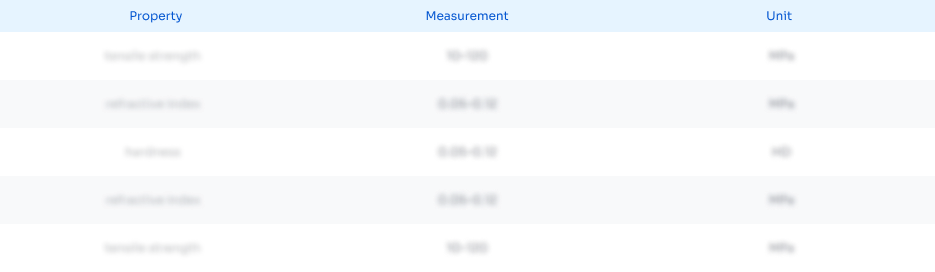
Abstract
Description
Claims
Application Information
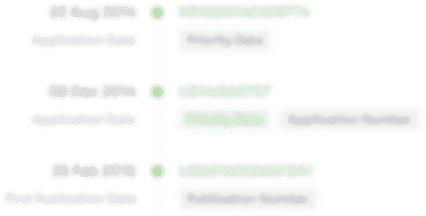
- R&D Engineer
- R&D Manager
- IP Professional
- Industry Leading Data Capabilities
- Powerful AI technology
- Patent DNA Extraction
Browse by: Latest US Patents, China's latest patents, Technical Efficacy Thesaurus, Application Domain, Technology Topic, Popular Technical Reports.
© 2024 PatSnap. All rights reserved.Legal|Privacy policy|Modern Slavery Act Transparency Statement|Sitemap|About US| Contact US: help@patsnap.com