Missing multi-modal representation learning algorithm for Alzheimer's disease diagnosis
An Alzheimer's disease and learning algorithm technology, applied in the field of missing multimodal representation learning algorithms, can solve problems such as missing multimodal data, and achieve the effect of good diagnosis accuracy and good development prospects.
- Summary
- Abstract
- Description
- Claims
- Application Information
AI Technical Summary
Problems solved by technology
Method used
Image
Examples
Embodiment Construction
[0021] The present invention will be further described in detail below in combination with specific embodiments. figure 1 A flow chart of the missing multimodal representation learning algorithm for Alzheimer's disease diagnosis of the present invention is given. Such as figure 1 As shown, the missing multimodal representation learning algorithm for Alzheimer's disease diagnosis of the present invention includes:
[0022] 1. Obtain the original data of MRI and PET-CT of Alzheimer's disease;
[0023] 2. Obtain the feature vector of each sample through data preprocessing;
[0024] 3. Use the autoencoder network to learn the potential representation of the complete MRI modality, while constraining the correlation between samples through graph regularization;
[0025] 5. Map the eigenvectors to the kernel space, fill in the missing information through kernel techniques, and constrain the correlation between the two modes through the Hilbert-Schmidt Independence Index (HSIC);
...
PUM
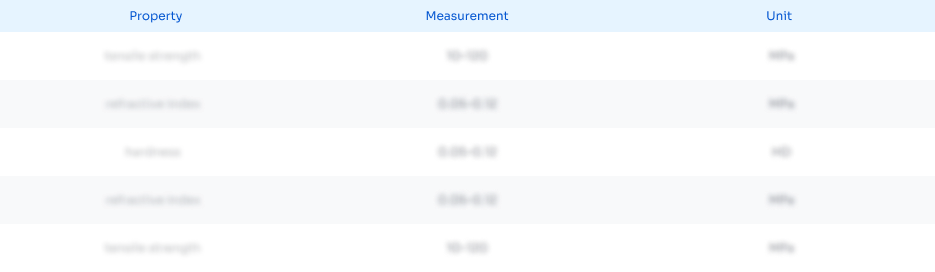
Abstract
Description
Claims
Application Information
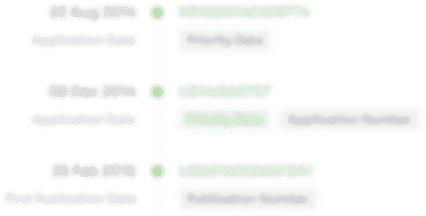
- R&D Engineer
- R&D Manager
- IP Professional
- Industry Leading Data Capabilities
- Powerful AI technology
- Patent DNA Extraction
Browse by: Latest US Patents, China's latest patents, Technical Efficacy Thesaurus, Application Domain, Technology Topic, Popular Technical Reports.
© 2024 PatSnap. All rights reserved.Legal|Privacy policy|Modern Slavery Act Transparency Statement|Sitemap|About US| Contact US: help@patsnap.com