2-exponential power deep neural network quantification method based on knowledge distillation training
A technology of deep neural network and quantization method, which is applied in the field of 2-exponential deep neural network quantization based on knowledge distillation training, can solve problems such as error and neural network accuracy loss of neural network, so as to reduce error, improve accuracy, and improve calculation. The effect of efficiency
- Summary
- Abstract
- Description
- Claims
- Application Information
AI Technical Summary
Problems solved by technology
Method used
Image
Examples
Embodiment Construction
[0026] The following will clearly and completely describe the technical solutions in the embodiments of the present invention with reference to the accompanying drawings in the embodiments of the present invention. Obviously, the described embodiments are only some, not all, embodiments of the present invention. Based on the embodiments of the present invention, all other embodiments obtained by persons of ordinary skill in the art without making creative efforts belong to the protection scope of the present invention.
[0027] Such as figure 1 As shown, the present invention provides a technical solution: a 2-exponential power deep neural network quantization method based on knowledge distillation training, including a teacher model and a 2-exponential power quantized student model, and the teacher network model has more parameters and a higher precision network The student model generally chooses a network model with fewer parameters and less precision than the teacher model...
PUM
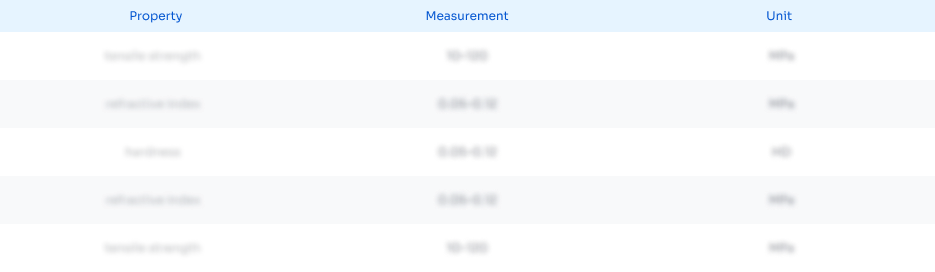
Abstract
Description
Claims
Application Information
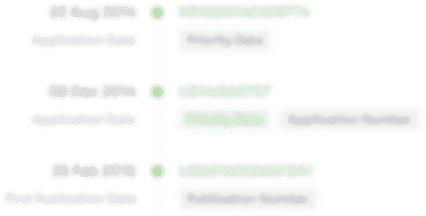
- R&D Engineer
- R&D Manager
- IP Professional
- Industry Leading Data Capabilities
- Powerful AI technology
- Patent DNA Extraction
Browse by: Latest US Patents, China's latest patents, Technical Efficacy Thesaurus, Application Domain, Technology Topic, Popular Technical Reports.
© 2024 PatSnap. All rights reserved.Legal|Privacy policy|Modern Slavery Act Transparency Statement|Sitemap|About US| Contact US: help@patsnap.com