Zero-sample target detection system and learnable semantic and fixed semantic fusion method
A target detection and sample technology, applied in the field of machine learning, can solve the problems of neural network training difficulties, weak identification ability, etc., and achieve the effect of improving accuracy
- Summary
- Abstract
- Description
- Claims
- Application Information
AI Technical Summary
Problems solved by technology
Method used
Image
Examples
Embodiment Construction
[0056] The application will be described in further detail below in conjunction with all the accompanying drawings.
[0057] The specific description of the zero-sample target detection problem is as follows: Assume that n tr visible classes and n ts Objects in unseen classes are detected, and the seen and unseen class spaces are disjoint. On the visible class space, given n tr A training set D labeled with target location and category information tr ={(b k , I k ,Y k ,a k ),k=1...n tr}, where b k is the kth label box, I k , Y k 、a k are the image, category label, and semantic attribute vector corresponding to the kth annotation box, respectively. while b k With a 4-tuple (x k ,y k ,w k ,h k ) to represent, where the first two elements x k and y k Indicates the coordinates of the upper left corner of the kth label box, and the last two values w k and h k are the width and height of the kth annotation box, respectively. Given a fixed category semantic ma...
PUM
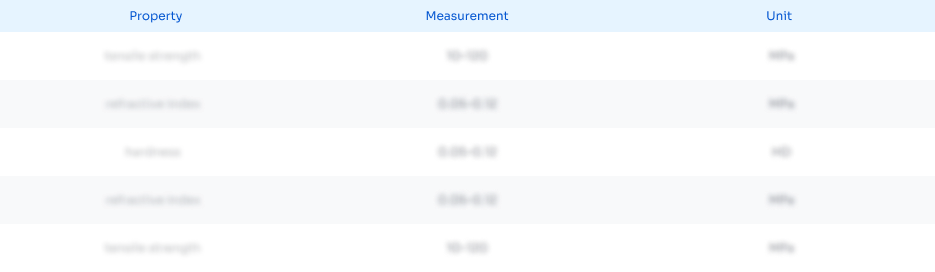
Abstract
Description
Claims
Application Information
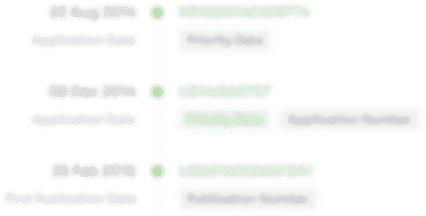
- R&D Engineer
- R&D Manager
- IP Professional
- Industry Leading Data Capabilities
- Powerful AI technology
- Patent DNA Extraction
Browse by: Latest US Patents, China's latest patents, Technical Efficacy Thesaurus, Application Domain, Technology Topic, Popular Technical Reports.
© 2024 PatSnap. All rights reserved.Legal|Privacy policy|Modern Slavery Act Transparency Statement|Sitemap|About US| Contact US: help@patsnap.com