Attribute network embedding and parameter-free clustering-based Bilibili user community discovery method
A discovery method and user technology, applied in the field of network science, can solve the problems of high complexity and low accuracy, and achieve the effect of improving the accuracy rate, reducing the dimension, and having a good application prospect.
- Summary
- Abstract
- Description
- Claims
- Application Information
AI Technical Summary
Problems solved by technology
Method used
Image
Examples
Embodiment Construction
[0027] The present invention will be further described below in conjunction with the accompanying drawings.
[0028] refer to Figure 1 ~ Figure 2 , a Bilibili user community discovery method based on attribute network embedding and non-parametric clustering, including the following steps:
[0029] Step 1: According to the existing Bilibili data, construct a user network model G=(V, E, F) with n nodes, V represents a node, E represents an edge, F represents an attribute, and each user is one Nodes, if there is concern between users, there will be edges. The edge relationship of n nodes forms an adjacency matrix A. The browsing history of each user is the attribute of the node. A total of m attributes are selected, and the attributes of all nodes Expressed as an attribute information matrix F with n rows and m columns;
[0030] Step 2: Transform the Bilibili user network G with n users and m attributes into n d-dimensional embedding vectors H in the feature space by using the...
PUM
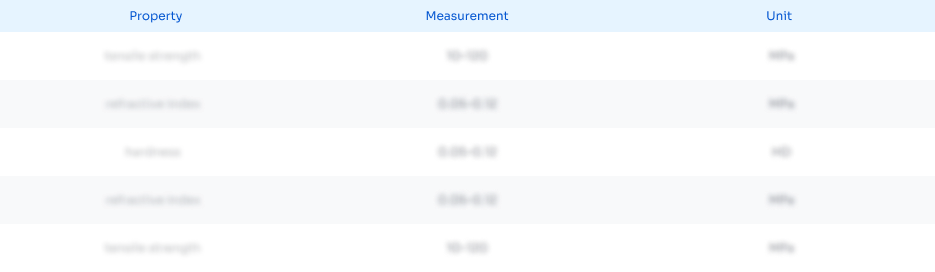
Abstract
Description
Claims
Application Information
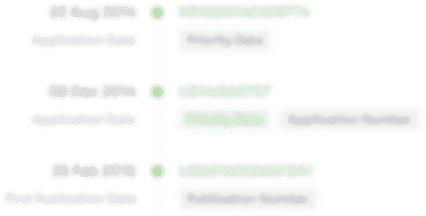
- R&D
- Intellectual Property
- Life Sciences
- Materials
- Tech Scout
- Unparalleled Data Quality
- Higher Quality Content
- 60% Fewer Hallucinations
Browse by: Latest US Patents, China's latest patents, Technical Efficacy Thesaurus, Application Domain, Technology Topic, Popular Technical Reports.
© 2025 PatSnap. All rights reserved.Legal|Privacy policy|Modern Slavery Act Transparency Statement|Sitemap|About US| Contact US: help@patsnap.com