Vehicle trajectory prediction method based on environmental attention neural network model
A neural network model and vehicle trajectory technology, which is applied in the field of vehicle trajectory prediction based on the environmental attention neural network model, can solve the problems that the environmental interaction features are not completely sufficient, and the extraction of environmental features is single, so as to achieve good trajectory prediction effect and improve accuracy. Effect of sex, effect improvement
- Summary
- Abstract
- Description
- Claims
- Application Information
AI Technical Summary
Problems solved by technology
Method used
Image
Examples
Embodiment Construction
[0014] The present invention will be further described below in conjunction with accompanying drawing.
[0015] Step1: Traffic scene modeling
[0016] The invention models the interaction relationship between the vehicles in the same traffic scene and the surrounding vehicles. For any vehicle in the traffic scene at each time t, the vehicle will interact with surrounding vehicles at the spatial position level, and the present invention uses an occupancy grid map to construct this spatial position structure. In addition, the feature information between vehicles will also be transmitted and updated in a non-Euclidean distance structure—a graph structure, so the present invention constructs this graph structure for transferring information between vehicles through nodes and edges.
[0017] 1. Initialize input features
[0018] In a static traffic scene at a certain moment, the basis for any behavior of the observed vehicle comes from two levels:
[0019] The first is to use th...
PUM
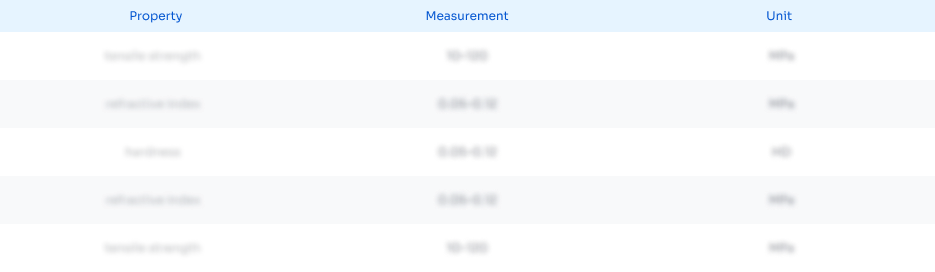
Abstract
Description
Claims
Application Information
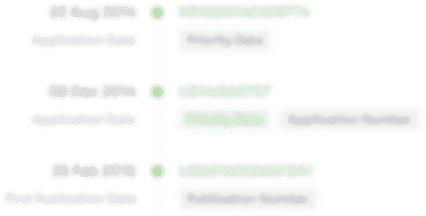
- R&D
- Intellectual Property
- Life Sciences
- Materials
- Tech Scout
- Unparalleled Data Quality
- Higher Quality Content
- 60% Fewer Hallucinations
Browse by: Latest US Patents, China's latest patents, Technical Efficacy Thesaurus, Application Domain, Technology Topic, Popular Technical Reports.
© 2025 PatSnap. All rights reserved.Legal|Privacy policy|Modern Slavery Act Transparency Statement|Sitemap|About US| Contact US: help@patsnap.com