Rolling bearing fault diagnosis method based on blind signal separation and support vector machine
A support vector machine and blind signal separation technology, applied in the testing of mechanical components, testing of machine/structural components, measuring devices, etc., can solve the problems of low diagnostic accuracy, poor robustness, and BP neural network easily falling into local extremum, etc. question
- Summary
- Abstract
- Description
- Claims
- Application Information
AI Technical Summary
Problems solved by technology
Method used
Image
Examples
Embodiment Construction
[0053] Below in conjunction with accompanying drawing and specific embodiment, the present invention is described in further detail:
[0054] refer to figure 1 , the present invention comprises the following steps:
[0055] Step 1) Obtain training sample set and test sample set:
[0056] Step 1a) Select 4 480 vibration time-domain signals containing different single fault type rolling bearings from the database, each rolling bearing has one or more vibration time-domain signals, and each vibration time-domain signal contains a single fault type of Fault type or non-fault type, select 120 vibration time-domain signals for each fault type, a total of 4 fault types rolling bearing vibration time-domain signals, label each vibration time-domain signal according to the fault type, and set the sample label of the rolling element fault is 1, the sample label of the inner ring fault is 2, the sample label of the outer ring fault is 3, and the sample label of no fault is 4. Combine e...
PUM
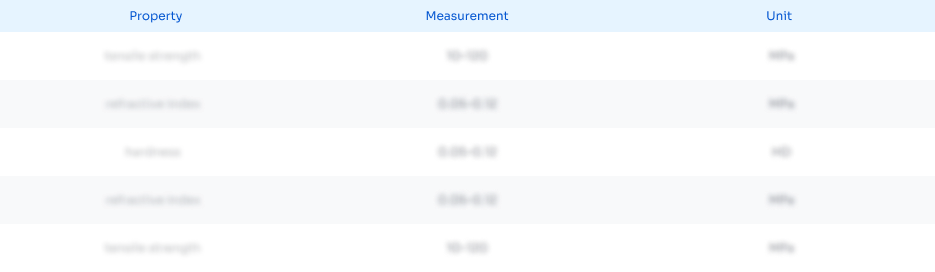
Abstract
Description
Claims
Application Information
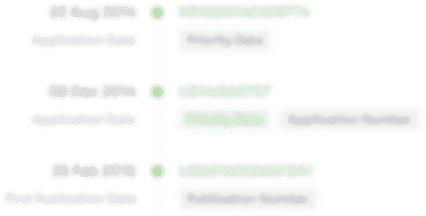
- R&D Engineer
- R&D Manager
- IP Professional
- Industry Leading Data Capabilities
- Powerful AI technology
- Patent DNA Extraction
Browse by: Latest US Patents, China's latest patents, Technical Efficacy Thesaurus, Application Domain, Technology Topic.
© 2024 PatSnap. All rights reserved.Legal|Privacy policy|Modern Slavery Act Transparency Statement|Sitemap