Convolutional neural network fault diagnosis method based on multi-channel attention module
A convolutional neural network and fault diagnosis technology, which is applied to biological neural network models, neural architectures, character and pattern recognition, etc., can solve the problems of poor generalization of convolutional neural network models, improve fault diagnosis accuracy, and suppress false Information, solve the effect of poor generalization performance
- Summary
- Abstract
- Description
- Claims
- Application Information
AI Technical Summary
Problems solved by technology
Method used
Image
Examples
Embodiment Construction
[0046] The present invention will be described in further detail below in conjunction with the accompanying drawings and specific embodiments. It should be understood that the specific embodiments described here are only used to explain the present invention, not to limit the present invention.
[0047] This embodiment means figure 1 The shown multi-channel attention module realizes the convolutional neural network fault diagnosis method; the bearing fault diagnosis under variable working conditions is an example, such as figure 2 As shown, it is an overall flowchart of a convolutional neural network bearing fault diagnosis method based on multi-channel attention module. The specific process is described as follows:
[0048] Step 1: Acquisition of bearing vibration signals, that is, using acceleration sensors to collect vibration signals of bearings under different working conditions;
[0049] Step 2: Perform data enhancement processing on the vibration signal collected in...
PUM
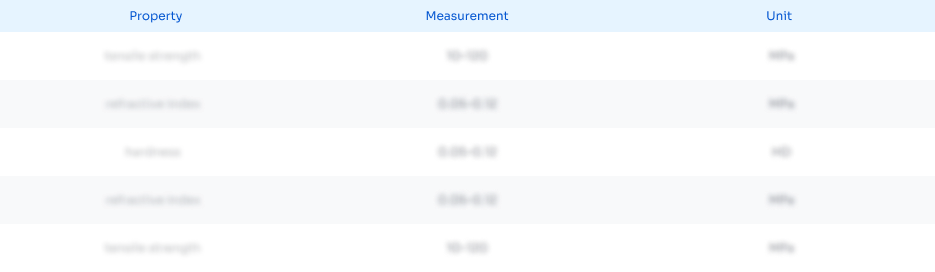
Abstract
Description
Claims
Application Information
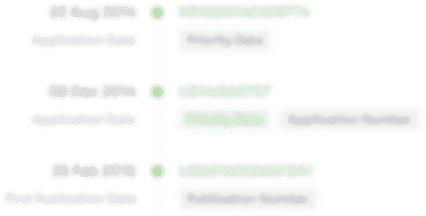
- R&D
- Intellectual Property
- Life Sciences
- Materials
- Tech Scout
- Unparalleled Data Quality
- Higher Quality Content
- 60% Fewer Hallucinations
Browse by: Latest US Patents, China's latest patents, Technical Efficacy Thesaurus, Application Domain, Technology Topic, Popular Technical Reports.
© 2025 PatSnap. All rights reserved.Legal|Privacy policy|Modern Slavery Act Transparency Statement|Sitemap|About US| Contact US: help@patsnap.com