Mixed granularity-based combined sparse method for neural network
A joint sparse and mixed granularity technology, applied in the engineering field, can solve problems such as low sparsity and low weight structure, and achieve the effect of ensuring model accuracy and reducing inference overhead.
- Summary
- Abstract
- Description
- Claims
- Application Information
AI Technical Summary
Problems solved by technology
Method used
Image
Examples
Embodiment Construction
[0030] The present invention will be described in further detail below in conjunction with the accompanying drawings and specific embodiments.
[0031] As shown in Figure 1(a), Figure 1(b) and Figure 1(c), the present invention proposes a joint sparse method based on mixed granularity for neural networks, which is used for image recognition, such as machine-readable For the automatic review of card test papers, first collect some image data and manually add labels to generate image data sets, which are divided into training data sets and test data sets; the training data sets are input into the convolutional neural network, and each part of the convolutional neural network is randomly initialized. The weight matrix of the layer is trained iteratively and the joint sparse process is used to prune the convolutional neural network; the test data set is used to cross-validate the training effect, and the weight matrix of each layer is updated through the back propagation algorithm ...
PUM
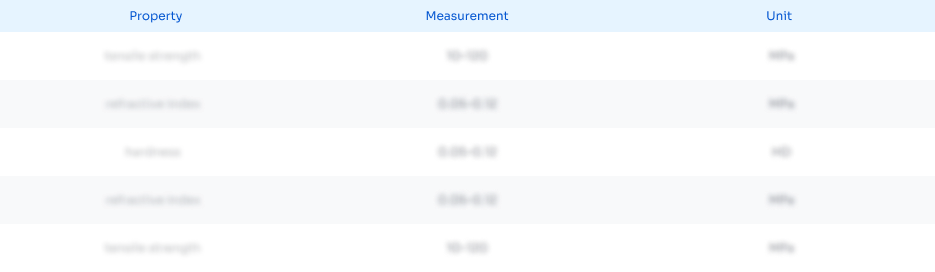
Abstract
Description
Claims
Application Information
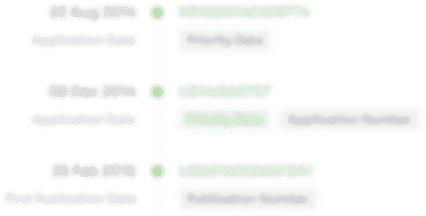
- R&D Engineer
- R&D Manager
- IP Professional
- Industry Leading Data Capabilities
- Powerful AI technology
- Patent DNA Extraction
Browse by: Latest US Patents, China's latest patents, Technical Efficacy Thesaurus, Application Domain, Technology Topic, Popular Technical Reports.
© 2024 PatSnap. All rights reserved.Legal|Privacy policy|Modern Slavery Act Transparency Statement|Sitemap|About US| Contact US: help@patsnap.com