Context-aware graph convolution recommendation system
A recommendation system and context technology, applied in biological neural network models, special data processing applications, instruments, etc., can solve problems such as time-consuming, high complexity, and inefficiency, and improve expression and generalization capabilities and test accuracy Improve the effect of less system parameters
- Summary
- Abstract
- Description
- Claims
- Application Information
AI Technical Summary
Problems solved by technology
Method used
Image
Examples
Embodiment Construction
[0015] The technical solutions in the embodiments of the present invention will be clearly and completely described below in conjunction with the accompanying drawings in the embodiments of the present invention. Obviously, the described embodiments are only some of the embodiments of the present invention, not all of them. Based on the embodiments of the present invention, all other embodiments obtained by persons of ordinary skill in the art without making creative efforts belong to the protection scope of the present invention.
[0016] The embodiment of the present invention provides a context-aware graph convolution recommendation system, which is a general recommendation system framework suitable for online services, which can not only combine various auxiliary information, but also capture the collaborative filtering effect to improve Model performance.
[0017] Such as figure 1 As shown, it is a schematic diagram of a context-aware graph convolution recommendation sys...
PUM
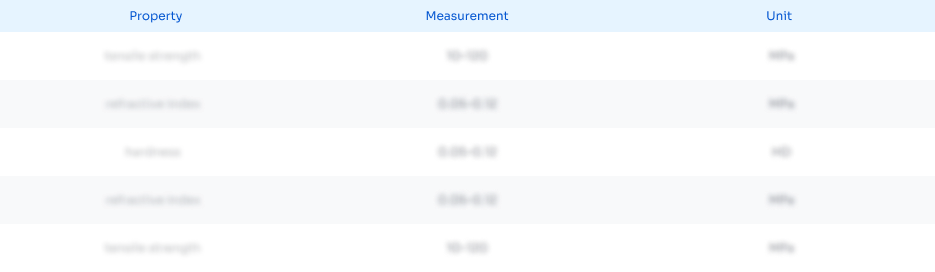
Abstract
Description
Claims
Application Information
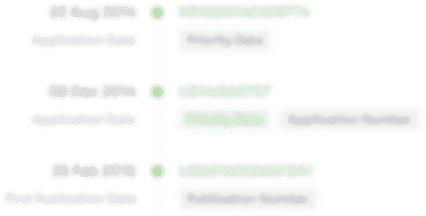
- R&D
- Intellectual Property
- Life Sciences
- Materials
- Tech Scout
- Unparalleled Data Quality
- Higher Quality Content
- 60% Fewer Hallucinations
Browse by: Latest US Patents, China's latest patents, Technical Efficacy Thesaurus, Application Domain, Technology Topic, Popular Technical Reports.
© 2025 PatSnap. All rights reserved.Legal|Privacy policy|Modern Slavery Act Transparency Statement|Sitemap|About US| Contact US: help@patsnap.com