Distributed deep learning multi-step delay updating method based on communication operation sparsification
A communication operation and deep learning technology, applied in neural learning methods, software deployment, biological neural network models, etc., can solve problems such as reducing the speed of distributed training, and achieve the effects of optimizing communication overhead, eliminating synchronization overhead, and reducing computing overhead.
- Summary
- Abstract
- Description
- Claims
- Application Information
AI Technical Summary
Problems solved by technology
Method used
Image
Examples
Embodiment Construction
[0025] In order to better understand the contents of the present invention, an example is given here.
[0026] The invention discloses a distributed deep learning multi-step delay update method (SSD-SGD) based on communication operation sparsification, and its specific steps include:
[0027] S1, warm-up training, use the synchronous stochastic gradient descent method to train the deep learning model for a certain number of iterations before performing multi-step delayed iterative training. The purpose is to make the weights and gradients of the network model tend to in a stable state.
[0028] S2, the switching phase, which only includes 2 iterations of training, which are used to complete the backup of the retrieved global weights and the first local parameter update operation respectively, the purpose of which is to switch the synchronous stochastic gradient descent update method to multi-step Delayed training mode. The local parameter update operation adopts the local up...
PUM
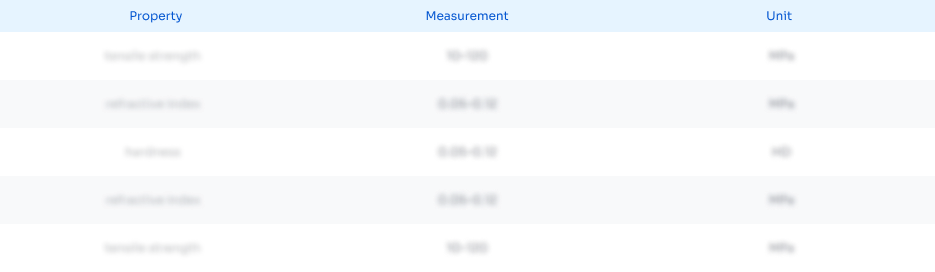
Abstract
Description
Claims
Application Information
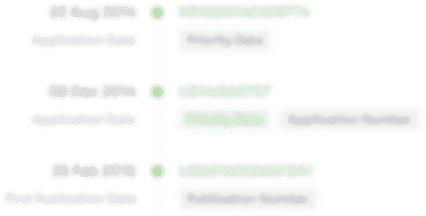
- R&D Engineer
- R&D Manager
- IP Professional
- Industry Leading Data Capabilities
- Powerful AI technology
- Patent DNA Extraction
Browse by: Latest US Patents, China's latest patents, Technical Efficacy Thesaurus, Application Domain, Technology Topic, Popular Technical Reports.
© 2024 PatSnap. All rights reserved.Legal|Privacy policy|Modern Slavery Act Transparency Statement|Sitemap|About US| Contact US: help@patsnap.com