Online household load power utilization combination identification and power consumption prediction method
A household load and load technology, which is applied in forecasting, neural learning methods, data processing applications, etc., can solve the problems of inability to real-time capture changes in the instantaneous process of household load combinations, weak scalability, and large online data acquisition errors. The effect of extensive and accurate combination forecast and improved forecast accuracy
- Summary
- Abstract
- Description
- Claims
- Application Information
AI Technical Summary
Problems solved by technology
Method used
Image
Examples
Embodiment Construction
[0047] In order to make the purpose, technical solution and advantages of the present invention clearer, the present invention will be further elaborated below in conjunction with the accompanying drawings.
[0048] In this example, see figure 1 As shown, the present invention proposes a method for online household load combination identification and power consumption prediction, including steps:
[0049] S10, collecting time-domain signals of monitoring data of total power and total current of electric loads based on time-series characteristics in real time through a non-intrusive data acquisition system;
[0050] S20, using Fourier transform and Laprado transform to perform dynamic time-frequency conversion on the time-domain signal of the load characteristic waveform, obtain a spectrum image using the load characteristic time-domain signal, and obtain total load data according to the spectrum images of all data;
[0051] S30. For the total load data, by extracting the eige...
PUM
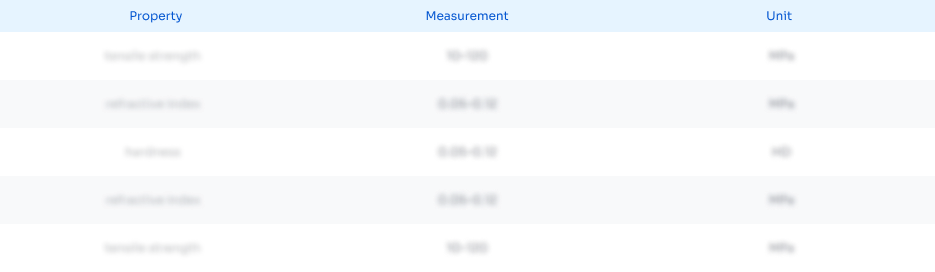
Abstract
Description
Claims
Application Information
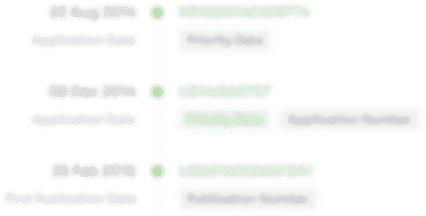
- R&D Engineer
- R&D Manager
- IP Professional
- Industry Leading Data Capabilities
- Powerful AI technology
- Patent DNA Extraction
Browse by: Latest US Patents, China's latest patents, Technical Efficacy Thesaurus, Application Domain, Technology Topic, Popular Technical Reports.
© 2024 PatSnap. All rights reserved.Legal|Privacy policy|Modern Slavery Act Transparency Statement|Sitemap|About US| Contact US: help@patsnap.com