Improved DCNN music genre classification method
A classification method and music technology, applied in speech analysis, neural learning method, electro-acoustic musical instruments, etc., can solve the problems of increasing model complexity and increasing the computational burden of model structure, and achieve obvious performance gain and simple calculation.
- Summary
- Abstract
- Description
- Claims
- Application Information
AI Technical Summary
Problems solved by technology
Method used
Image
Examples
Embodiment Construction
[0034]The specific implementation of the present invention will be described in detail below in conjunction with the accompanying drawings. As a part of this specification, the principles of the present invention will be described through examples. Other aspects, features and advantages of the present invention will become clear through the detailed description. In the referenced drawings, the same reference numerals are used for the same or similar components in different drawings.
[0035] Such as figure 1 Shown, the music genre classification method of improved DCNN of the present invention is characterized in that, comprises the following steps:
[0036] Step 1: Input training set and verification set;
[0037] Step 2: Extract audio information MFCC features;
[0038] Step 3: generate spectrum;
[0039] Step 4: Spectrum cutting;
[0040] Step 5: Input the network model;
[0041] Step 6: Train the model;
[0042] Step 7: Verify the model;
[0043] Step 8: Whether the...
PUM
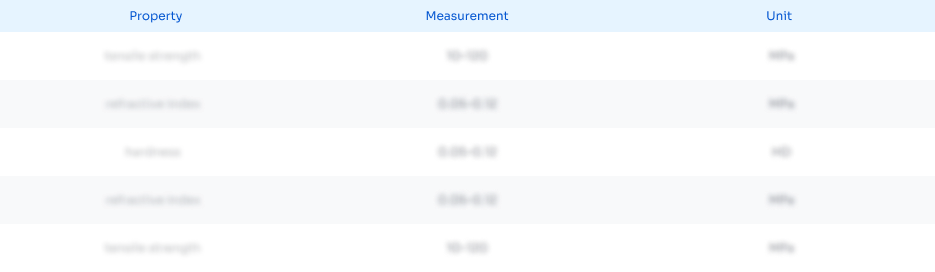
Abstract
Description
Claims
Application Information
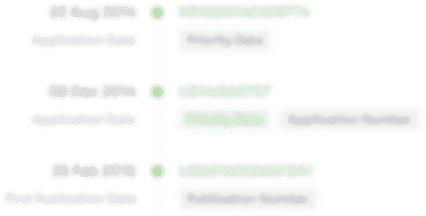
- R&D Engineer
- R&D Manager
- IP Professional
- Industry Leading Data Capabilities
- Powerful AI technology
- Patent DNA Extraction
Browse by: Latest US Patents, China's latest patents, Technical Efficacy Thesaurus, Application Domain, Technology Topic, Popular Technical Reports.
© 2024 PatSnap. All rights reserved.Legal|Privacy policy|Modern Slavery Act Transparency Statement|Sitemap|About US| Contact US: help@patsnap.com