Quantitative statistical characterization method of aluminum alloy micron-scale second phase based on deep learning
A deep learning and aluminum alloy surface technology, applied in the field of quantitative statistical representation of aluminum alloy micron-level second phase based on deep learning, can solve the problems of full field of view and inter-partition distribution differences, single characterization parameters, etc., and achieve a large field of view , complete information, avoid the effect of manual marking
- Summary
- Abstract
- Description
- Claims
- Application Information
AI Technical Summary
Problems solved by technology
Method used
Image
Examples
Embodiment Construction
[0051] The following will clearly and completely describe the technical solutions in the embodiments of the present invention with reference to the accompanying drawings in the embodiments of the present invention. Obviously, the described embodiments are only some, not all, embodiments of the present invention. Based on the embodiments of the present invention, all other embodiments obtained by persons of ordinary skill in the art without making creative efforts belong to the protection scope of the present invention.
[0052] The purpose of the present invention is to provide a quantitative statistical characterization method for micron-level second phases of aluminum alloys based on deep learning, which uses high-throughput image data acquisition methods and deep learning algorithms to realize automatic identification of micron-level second phases of aluminum alloys, and combines The mathematical method excavates various characterization parameters of the second phase after ...
PUM
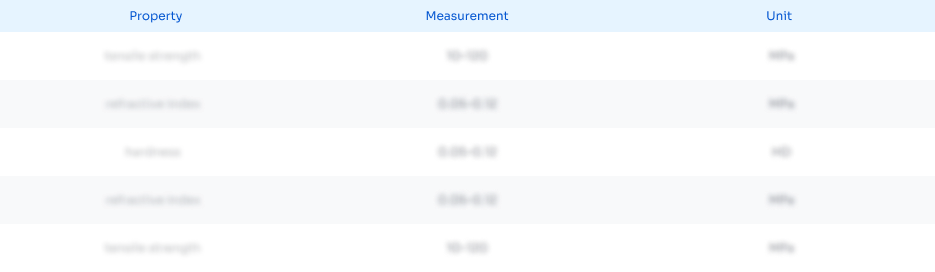
Abstract
Description
Claims
Application Information
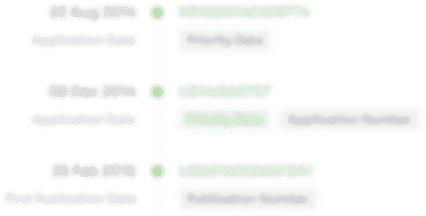
- R&D Engineer
- R&D Manager
- IP Professional
- Industry Leading Data Capabilities
- Powerful AI technology
- Patent DNA Extraction
Browse by: Latest US Patents, China's latest patents, Technical Efficacy Thesaurus, Application Domain, Technology Topic, Popular Technical Reports.
© 2024 PatSnap. All rights reserved.Legal|Privacy policy|Modern Slavery Act Transparency Statement|Sitemap|About US| Contact US: help@patsnap.com