Rolling bearing fault diagnosis method based on enhanced lightweight multi-scale CNN
A rolling bearing and fault diagnosis technology, which is applied in neural learning methods, measuring devices, and testing of mechanical components, can solve problems such as insignificant time scale diversity, economical degradation of rotating machinery, and wear and tear of rolling bearings, and achieve enhanced discriminative fault features Extraction ability, improvement of practical application value, effect of less parameters
- Summary
- Abstract
- Description
- Claims
- Application Information
AI Technical Summary
Problems solved by technology
Method used
Image
Examples
Embodiment Construction
[0036] The specific embodiments of the present invention will be described below in conjunction with the accompanying drawings.
[0037] Such as figure 1 As shown, a rolling bearing fault diagnosis method based on an enhanced lightweight multi-scale CNN of the present embodiment includes the following steps:
[0038] Step S1: performing multi-dimensional feature extraction on the original vibration signal data;
[0039] Step S2: Extract rich and complementary multi-scale features through a lightweight multi-scale network, and use a discriminative fault feature enhancement mechanism (DFRM) to screen and enhance the extracted multi-scale features;
[0040] Step S3: Perform multi-level and multi-scale feature fusion through layer-skip connection;
[0041] Step S4: Repeat steps S2 and S3 several times to extract high-level abstract features of the original vibration signal;
[0042] Step S5: classifier training, fault identification.
[0043] The specific implementation is as ...
PUM
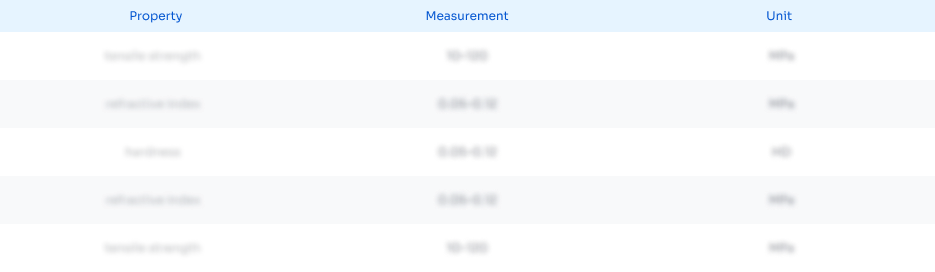
Abstract
Description
Claims
Application Information
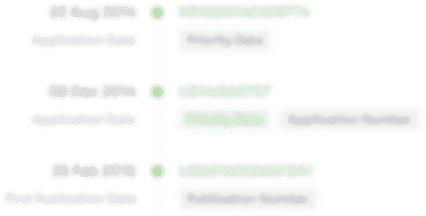
- R&D Engineer
- R&D Manager
- IP Professional
- Industry Leading Data Capabilities
- Powerful AI technology
- Patent DNA Extraction
Browse by: Latest US Patents, China's latest patents, Technical Efficacy Thesaurus, Application Domain, Technology Topic, Popular Technical Reports.
© 2024 PatSnap. All rights reserved.Legal|Privacy policy|Modern Slavery Act Transparency Statement|Sitemap|About US| Contact US: help@patsnap.com