Deep learning-based automatic detection method and system for multiple growth periods of rice ears
A technology of deep learning and automatic detection, applied in neural learning methods, instruments, biological neural network models, etc., can solve problems such as limited detection and inaccuracy
- Summary
- Abstract
- Description
- Claims
- Application Information
AI Technical Summary
Problems solved by technology
Method used
Image
Examples
Embodiment 1
[0049] Such as figure 1 As shown, a deep learning-based automatic detection method for multiple developmental stages of rice ears, including the following steps:
[0050] S1: Construct and train a target detection network based on deep learning to obtain a target detection model;
[0051] S2: Online continuous collection of n-day image data in the monitoring area;
[0052] S3: The image data is used as the input of the target detection model, and the number of various rice ears with different maturity levels in each image is detected;
[0053] S4: Calculate the average value of the number of various rice ears in all image data collected in the i-th day as the average number of rice ears of each type on that day;
[0054] S5: Calculate the density of various rice ears in the image according to the area of the monitoring area and the daily average number of rice ears of each type;
[0055] S6: Judging whether the density of various rice ears has increased significantly and ...
Embodiment 2
[0066] More specifically, on the basis of Example 1, in order to solve the problem that other image sequence-based automatic detection methods for rice ear development are only applicable to a certain key development stage of rice ears, a deep learning-based method is provided. The automatic detection method of multiple developmental stages of rice ears can use ground monitoring equipment to acquire rice image sequences in real time to accurately detect which developmental stage rice is in, and has good applicability for different varieties of rice in different scenarios. Specifically:
[0067] First, based on the crop big data collection platform, the image data of rice from earing to harvest is obtained. Preferably, the images are taken at an angle close to the vertical, and the collected real-time front and bottom images are taken as the research object. Note that it is necessary to measure the actual area S of the monitoring area of the camera on the spot. It is also pos...
Embodiment 3
[0074] More specifically, the present invention will be described in further detail below with reference to the examples and accompanying drawings, but the embodiments of the present invention are not limited thereto.
[0075] The camera deployment strategy of the present invention is as attached figure 2 , Jinnong silk seedling rice is planted in a square field of about 40m×40m, with 2 to 3 seedlings in each hole, and the hole interval is about 15-18cm. In the experiment, four identical cameras were arranged in the four corners of the field for rice monitoring. The cameras used Hikvision DS-2DC7423IW-A series smart ball cameras, fixed at a height of 2.5m from the ground, and the field of view of the lenses was the same. The actual area of the monitoring area is S.
[0076] The present invention is based on deep learning to realize the automatic detection method of multiple developmental stages of rice ears, and the overall technical flow chart of the method is as attached...
PUM
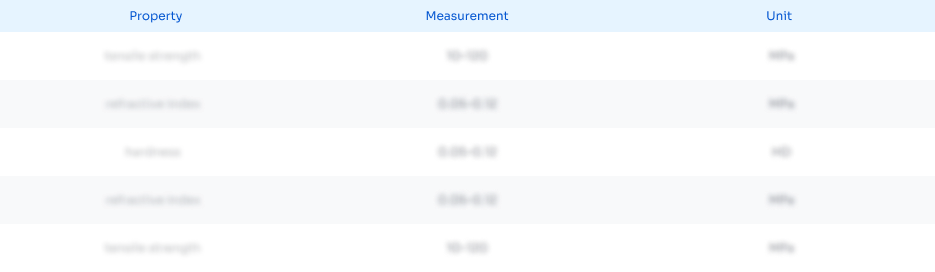
Abstract
Description
Claims
Application Information
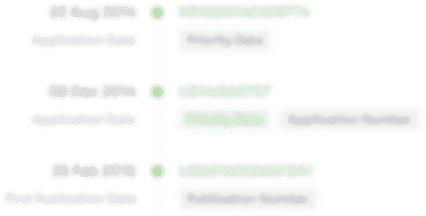
- R&D Engineer
- R&D Manager
- IP Professional
- Industry Leading Data Capabilities
- Powerful AI technology
- Patent DNA Extraction
Browse by: Latest US Patents, China's latest patents, Technical Efficacy Thesaurus, Application Domain, Technology Topic, Popular Technical Reports.
© 2024 PatSnap. All rights reserved.Legal|Privacy policy|Modern Slavery Act Transparency Statement|Sitemap|About US| Contact US: help@patsnap.com