Pulmonary nodule recognition and segmentation method and system based on deep learning
A technology of deep learning and pulmonary nodules, applied in the field of medical image processing, can solve problems such as huge training parameters, slow prediction speed, and difficulty in convergence, and achieve high generalization performance, fast speed, and improved sensitivity
- Summary
- Abstract
- Description
- Claims
- Application Information
AI Technical Summary
Problems solved by technology
Method used
Image
Examples
Embodiment Construction
[0034] In order to make the object, technical solution and advantages of the present invention clearer, the present invention will be further described in detail below in conjunction with the accompanying drawings.
[0035] see figure 1 , figure 1 Shows the flow chart of an embodiment of the deep learning-based pulmonary nodule recognition and segmentation method of the present invention, which includes:
[0036] S101, preprocessing the DICOM file to generate a chest CT image, segmenting a lung mask from the chest CT image, and patching the contour of the lung mask.
[0037] DICOM (Digital Imaging and Communications in Medicine) is an international standard (ISO 12052) for medical images and related information. It defines a medical image format that can be used for data exchange with a quality that meets clinical needs.
[0038] It should be noted that by preprocessing the DICOM file, the real CT value of the CT image in the DICOM file can be effectively restored, and the ...
PUM
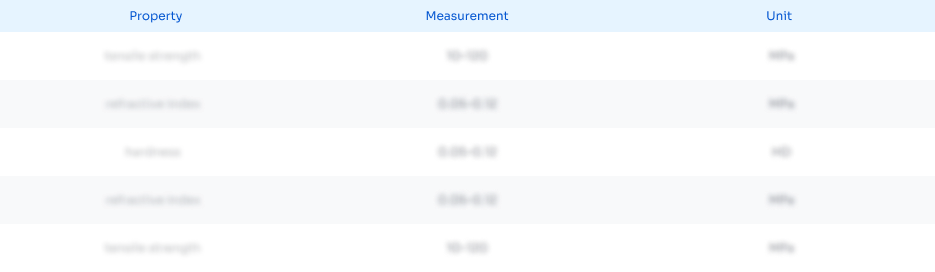
Abstract
Description
Claims
Application Information
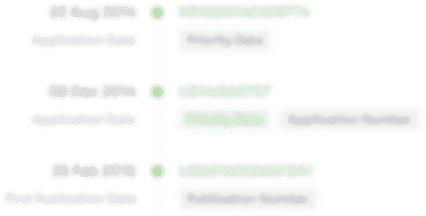
- R&D Engineer
- R&D Manager
- IP Professional
- Industry Leading Data Capabilities
- Powerful AI technology
- Patent DNA Extraction
Browse by: Latest US Patents, China's latest patents, Technical Efficacy Thesaurus, Application Domain, Technology Topic, Popular Technical Reports.
© 2024 PatSnap. All rights reserved.Legal|Privacy policy|Modern Slavery Act Transparency Statement|Sitemap|About US| Contact US: help@patsnap.com