Data augmentation method based on RBSAGAN
A technology of data and quantity, applied in the field of using deep learning method to generate EEG signals of motor imagery, can solve the problems of missing features, limited feature information of EEG signals, not making full use of signal timing features, etc. Characteristic information is not comprehensive, and the effect of change is realized
- Summary
- Abstract
- Description
- Claims
- Application Information
AI Technical Summary
Problems solved by technology
Method used
Image
Examples
Embodiment Construction
[0028] The experiment of the present invention is carried out in the following hardware environment: Intel Xeon E5-2683 2.00Hz CPU with 14 cores and GeForce 1070GPU with 8GB memory. All neural networks are implemented using the Pytorch framework.
[0029] The data used in the present invention is the "BCI Competition IV 2a" public data set. The EEG signals were collected by a 22-conductor electrode cap with a specification of 10-20 system and a sampling frequency of 250 Hz. Nine subjects performed four types of motor imagery tasks: left hand, right hand, foot, tongue. Each subject conducts two days of experiments, each day contains 288 experiments, a total of 576 experiments. EEG signals were filtered through a band-pass filter from 0.5 Hz to 100 Hz and a notch filter at 50 Hz. Arrow indications appeared at 2s in each experiment, and the direction was left, right, up or down (corresponding to one of the four types of tasks left hand, right hand, tongue or foot), and kept fo...
PUM
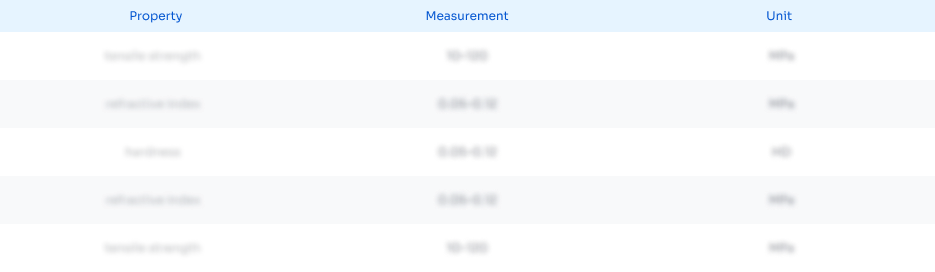
Abstract
Description
Claims
Application Information
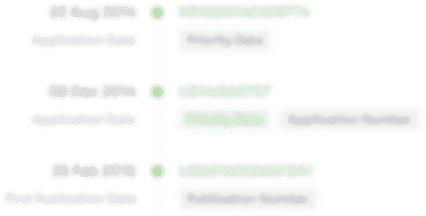
- R&D
- Intellectual Property
- Life Sciences
- Materials
- Tech Scout
- Unparalleled Data Quality
- Higher Quality Content
- 60% Fewer Hallucinations
Browse by: Latest US Patents, China's latest patents, Technical Efficacy Thesaurus, Application Domain, Technology Topic, Popular Technical Reports.
© 2025 PatSnap. All rights reserved.Legal|Privacy policy|Modern Slavery Act Transparency Statement|Sitemap|About US| Contact US: help@patsnap.com